SPIEGEL ONLINE: AMA with Christina Elmer, Marcel Pauly, and Patrick Stotz
Conversations with Data: #18
Do you want to receive Conversations with Data? Subscribe
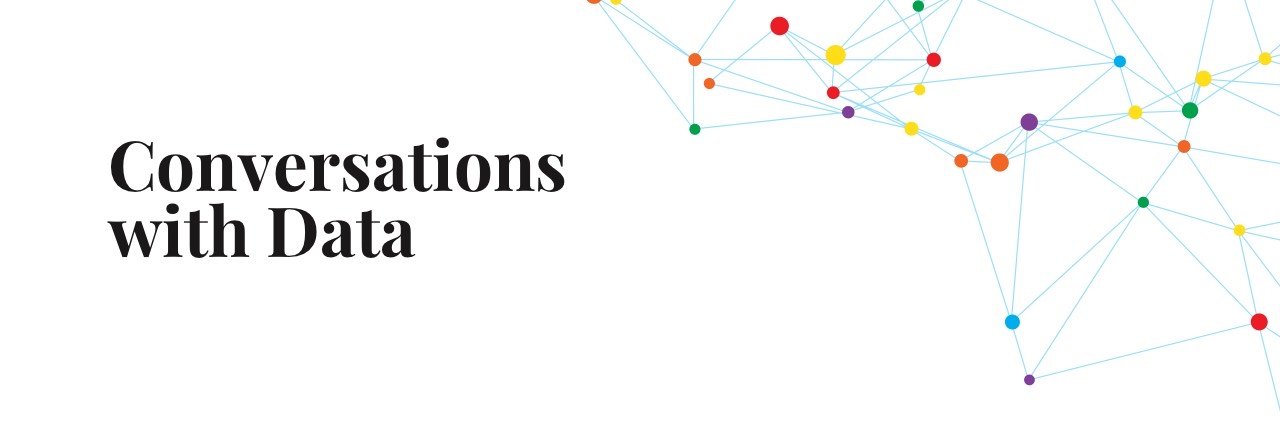
What do Google, Facebook, and a German credit bureau have in common? As it turns out, a lot. At least when it comes to operating seemingly harmless algorithms.
At SPIEGEL ONLINE, they’ve taken each of these companies on in a major drive to put algorithms in the spotlight and hold their developers responsible. But with 6 million Google search results, 600 Facebook political ads, and 16,000 credit reports to trawl through, these investigations are no easy feat.
So how do they do it? The answer, they’ve told us, is collaboration.
Welcome to our 18th edition of Conversations with Data, where we have Christina Elmer, Marcel Pauly, and Patrick Stotz with us from SPIEGEL ONLINE’s data team to answer your questions on algorithmic accountability reporting, and the teamwork behind it.
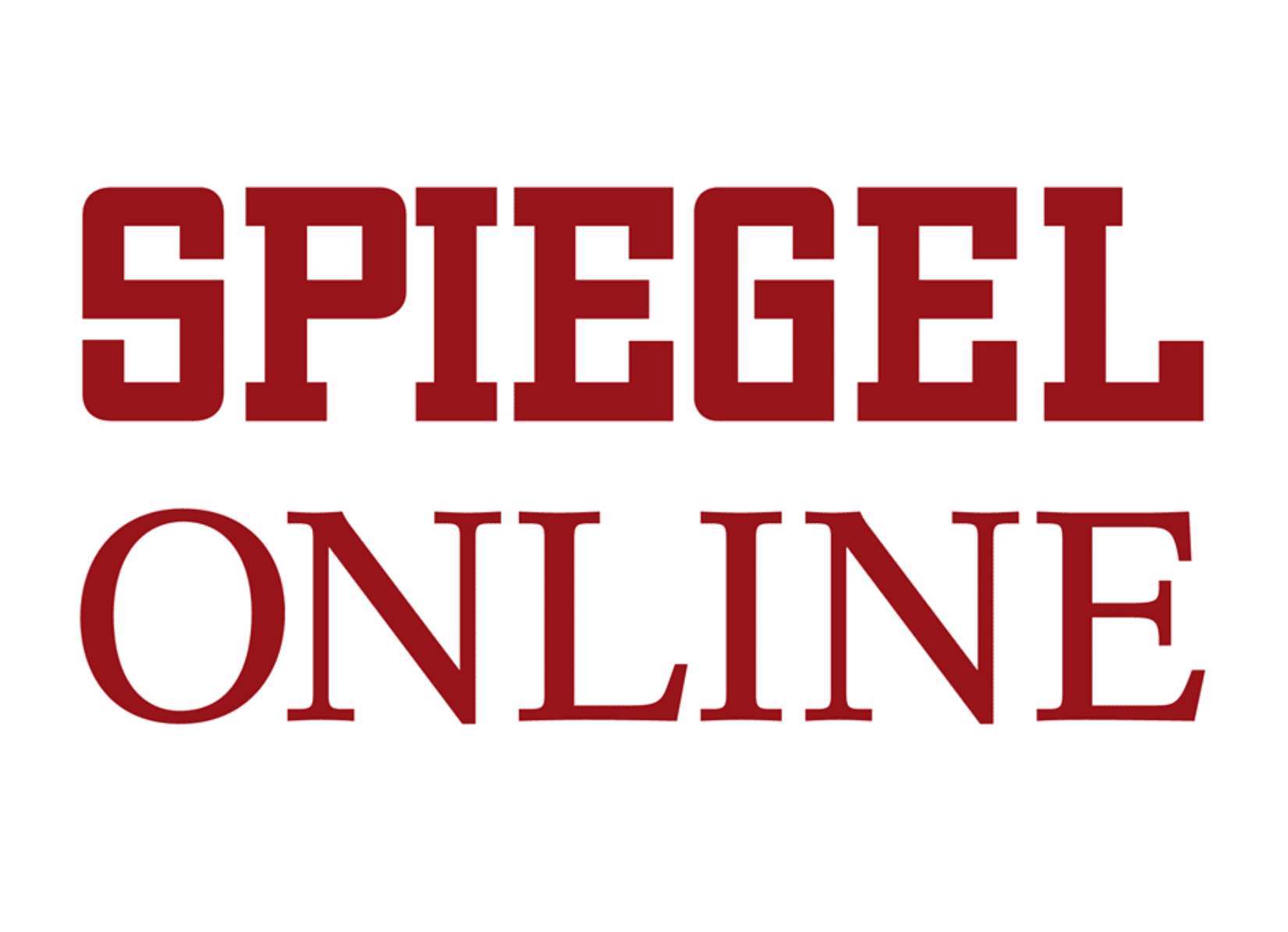
What you asked
As we’ve mentioned, investigating algorithms isn’t for the fainthearted -- something you were also keen to hear about when you asked:
What’s the most challenging aspect of reporting on algorithms?
Christina: “Many of the algorithms we want to investigate are like black boxes. In many cases it is impossible to understand exactly how they work, because as journalists we often cannot analyse them directly. This makes it difficult to substantiate hypotheses and verify stories. In these cases, any mechanism we can make transparent is very valuable. In any case, however, it seems advisable to concentrate on the impact of an algorithm and to ask what effect it has on society and individuals. The advantage of this: from the perspective of our readers, these are the more relevant questions anyway.”
You mentioned looking at impacts -- algorithmic accountability reporting often uncovers unintended negative impacts, have you ever discovered any unexpected positive effects?
Marcel: “We haven't discovered any unexpected positive effects by algorithms, yet. But generally speaking, from an investigating perspective, it can be considered positive that decisions made by algorithms are more comprehensible and reproducible, once you know the decision criteria. Human decisions are potentially even less transparent.”
So, what kinds of skills should newsrooms invest in to report on this beat?
Christina: “There should be a permanent team of data journalists or at least editorial data analysts. It needs this interface between journalism, analysis and visualisation to have the relevant skills for algorithmic accountability reporting on board and to permanently keep them up to date. In addition, these teams are perfect counterparts to external cooperation partners, who often play an important role in such projects.”
Let’s talk some more about the team’s collaborations. Does the team engage external specialists to understand the complexities of certain algorithms?
Marcel: “When we investigated the credit bureau Schufa's scoring algorithm we teamed up with our colleagues from Bayerischer Rundfunk. We were only a couple of (data) journalists and a data scientist, so of course we consulted practitioners like bank insiders who have worked with the algorithm's output. And we asked data and consumer protection advocates about their experiences with Schufa scoring. Only in this way do you get a deeper understanding of the matter you're reporting on.”
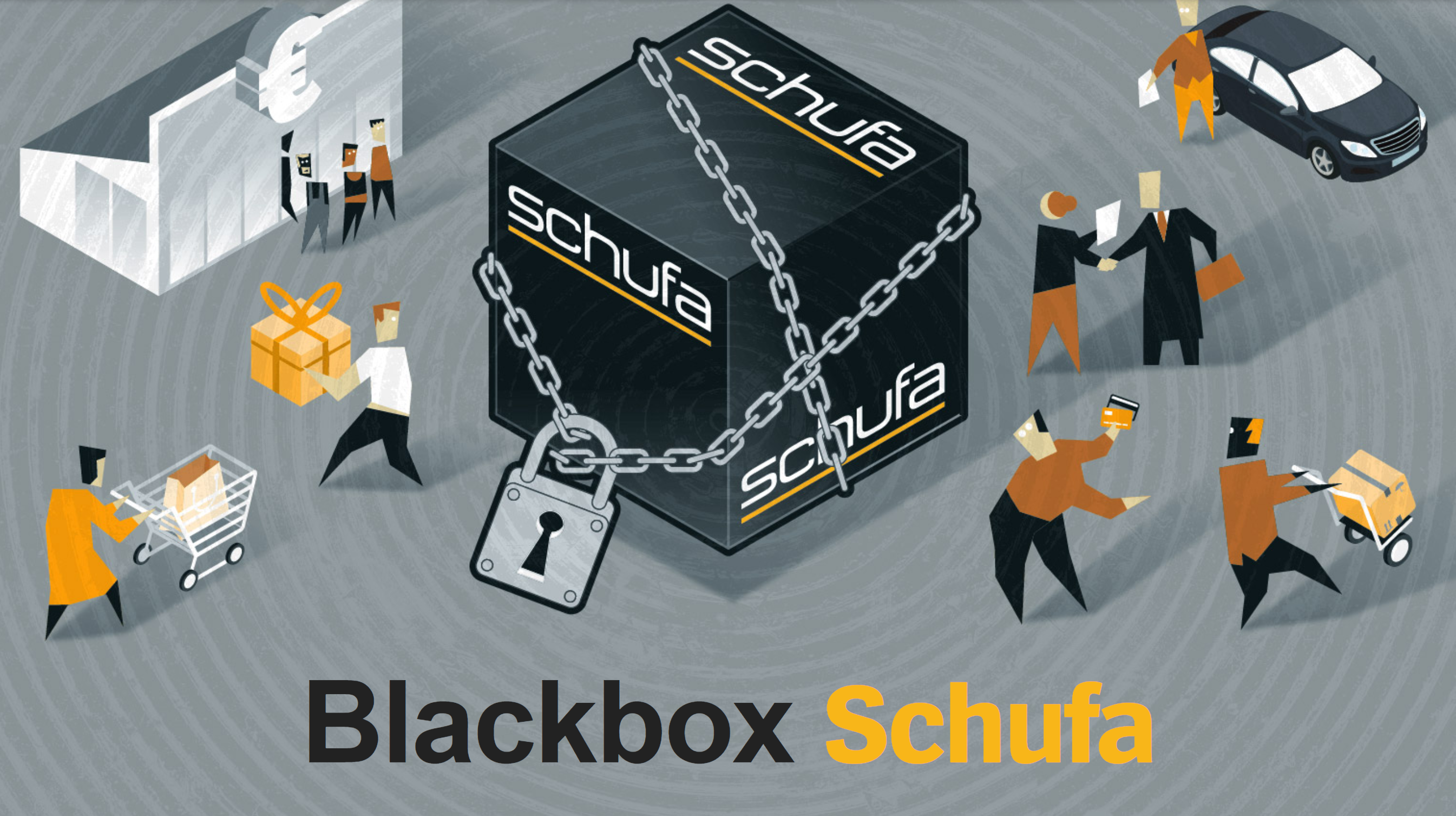
Image: SPIEGEL ONLINE.
What about internally? How does your team collaborate with other teams at SPIEGEL ONLINE?
Patrick: “In our workflow, the collaboration between data journalists and editors covering a certain beat is routine practice. However, algorithmic accountability projects come with an extra challenge for ‘ordinary reporters’. Most of the time, they won’t be able to actively participate in the technical part of the investigation and will rely on our work. As a result, we in our team have to develop a deeper understanding of the specific domain than in most other data journalistic projects. At the same time, constant exchange with colleagues that have domain specific knowledge should never be neglected. Our strategy is to establish a constant back and forth between us investigating and them commenting results or pushing us into the right direction. We try to explain and get them involved as much as possible but do realise that it’s on us to take responsibility for the core of the analysis.”
Christina, you’ve written a chapter in the Data Journalism Handbook 2 on collaborative investigations. If there was only one tip you would like readers to take from your chapter, what would it be?
Christina: “Don't dream of completely deconstructing an algorithm. Instead, put yourself in the perspective of your audience and think about the effects that directly affect their lives. Follow these approaches and hypotheses – preferably in collaboration with other teams and experts. This leads to better results, a greater impact and much more fun.”
Our next conversation
From an emerging issue, to something a little bit older. Next time, we’ll be featuring your favourite examples of historical data journalism.
It’s a common misconception that data reporting has only evolved in tandem with computers. But reporting with data has existed long before new technologies. You need only look at the charts featured in the New York Times throughout the 1800s, or The Economist’s front-page table in its inaugural 1843 edition.
Until next time,
Madolyn from the EJC Data team
Time to have your say
Sign up for our Conversations with Data newsletter
Join 10.000 data journalism enthusiasts and receive a bi-weekly newsletter or access our newsletter archive here.
Almost there...
If you experience any other problems, feel free to contact us at [email protected]