Algorithmic reporting
Conversations with Data: #2
Do you want to receive Conversations with Data? Subscribe
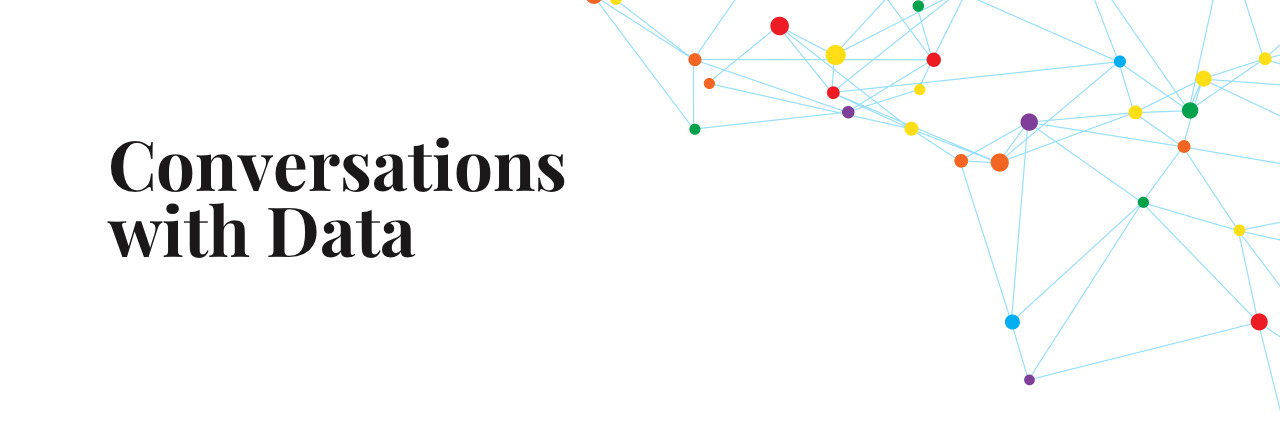
Hello, data enthusiasts!
Welcome to the second edition of the European Journalism Centre's data newsletter.
Each edition, we’ll be opening up a conversation about data with you. We know that the best way to master data journalism is by doing, which means you’re in the best position to help others grow from your mistakes and learn your triumphs.
To kick off the conversation, we asked you to submit your experiences reporting with algorithms. We also gave you the opportunity to ask Nick Diakopoulous, one of our Data Journalism Handbook authors, questions on the subject.
What you said
For those of you using automation, your advice focused on the importance of defining purpose. While automation projects have the potential to find and produce stories on underreported data, these can be overshadowed by gimmicky uses of technology.
Jens Finnäs, from Newsworthy, submitted: "automation is not - or should not - only be about technology. With an engineer mindset it is tempting to develop automated solutions because you can, rather than because someone asked you to. Going forward we are taking influence from design thinking and asking ourselves what real problems can we solve."
Laurence Dierickx agreed that, before undertaking an automation project, journalists should ask themselves: "what is the added value in terms of journalistic purpose?" Once this is defined, you should consider whether a project is suitable by assessing your data’s quality, reliability, and relevance to your story.
Even if your data doesn’t meet these standards, you might still have a chance at finding a story. "If data are a total mess, this could also tell you a lot about the management of the data producer (and a good story could be hidden behind)," she said.
What you asked
Now to reporting on algorithms.
In his Data Journalism Handbook chapter, The Algorithms Beat, Nick looks at the emerging practice of algorithmic accountability reporting. For this beat, the traditional watchdog role of journalists is focused on holding powerful algorithms to account. Think ProPublica’s Machine Bias series or Gizmodo’s coverage of Facebook’s PYMK algorithm.
But, you asked, how does this compare to traditional watchdog reporting?
Nick: "Watchdogging algorithmic power is conceptually quite similar to watchdogging other powerful actors in society. With algorithms you’re looking out for wrongdoings like discriminatory decisions, individually or societally impactful errors, violations of laws or social norms, or in some cases misuse by the people operating the algorithmic system."
So, what’s the difference?
Nick: "What’s different is that algorithms can be highly technical and involve machine learning methods that themselves can be difficult to explain, they can be difficult to gain access to because they’re behind organisational boundaries and often can’t be compelled through public records requests, and they’re capricious and can change on a dime."
Does this mean that non-data journalists can’t investigate algorithms?
Nick: "Non-data journalists can absolutely participate in algorithmic accountability reporting! Daniel Trielli and I wrote a piece last year for Columbia Journalism Review that outlines some ways, like looking at who owns an algorithm and how it’s procured, as well as reporting on the scale and magnitude of potential impact."
Read Nick's full answers here.
Our next conversation
Well, that’s it for algorithms.
We learnt a lot crowdsourcing your advice and questions for our first conversation. This got us thinking about the challenges and opportunities of using crowdsourced data in more complex reporting projects—which brings us to the topic of our next conversation.
That’s right, we’re going to be crowdsourcing your thoughts on crowdsourcing.
If you’ve ever asked the crowd for data, worked with crowdsourced data, or even submitted data as part of the crowd, we want to hear from you!
Until next time,
Madolyn from the EJC Data team
Time to have your say
Sign up for our Conversations with Data newsletter
Join 10.000 data journalism enthusiasts and receive a bi-weekly newsletter or access our newsletter archive here.
Almost there...
If you experience any other problems, feel free to contact us at [email protected]