6. How to think about deepfakes and emerging manipulation technologies
Written by: Sam Gregory
Sam Gregory is program director of WITNESS (www.witness.org), which helps people use video and technology to fight for human rights. An award-winning technologist and advocate, he is an expert on new forms of AI-driven mis/disinformation and leads work around emerging opportunities and threats to activism and journalism. He is also co-chair of the Partnership on AI’s expert group focused on AI and the media.
In the summer of 2018, Professor Siwei Lyi, a leading deepfakes researcher based at the University of Albany, released a paper showing that deepfake video personas did not blink at the same rate as real people. This claim was soon covered by Fast Company, New Scientist, Gizmodo, CBS News and others, causing many people to come away thinking they now had a robust way of spotting a deepfake.
Yet within weeks of publishing his paper, the researcher received videos showing a deepfake persona blinking like a human. As of today, this tip isn’t useful or accurate. It was the Achilles’ heel of a deepfakes creation algorithm at that moment, based on the training data being used. But within months it was no longer valid.
This illustrates a key truth about deepfake detection and verification: Technical approaches are useful until synthetic media techniques inevitably adapt to them. A perfect deepfake detection system will never exist.
So how should journalists verify deepfakes, and other forms of synthetic media?
The first step is to understand the cat-and-mouse nature of this work and be aware of how the technology is evolving. Second, journalists need to learn and apply fundamental verification techniques and tools to investigate whether a piece of content has been maliciously manipulated or synthetically generated. The approaches to image and video verification detailed in the first Verification Handbook, as well as in First Draft’s resources related to visual verification all apply. Finally, journalists need to understand we’re already in an environment where falsely claiming that something is a deepfake is increasingly common. That means the ability to verify a photo or video’s authenticity is just as important as being able to prove it has been manipulated.
This chapter expands on these core approaches to verifying deepfakes, but it’s first important to have a basic understanding of deepfakes and synthetic media.
What are deepfakes and synthetic media?
Deepfakes are new forms of audiovisual manipulation that allow people to create realistic simulations of someone’s face, voice or actions. They enable people to make it seem like someone said or did something they didn’t. They are getting easier to make, requiring fewer source images to build them, and they are increasingly being commercialized. Currently, deepfakes overwhelmingly impact women because they’re used to create nonconsensual sexual images and videos with a specific person’s face. But there are fears deepfakes will have a broader impact across society and in newsgathering and verification processes.
Deepfakes are just one development within a family of artificial intelligence (AI)-enabled techniques for synthetic media generation. This set of tools and techniques enable the creation of realistic representations of people doing or saying things they never did, realistic creation of people/objects that never existed, or of events that never happened.
Synthetic media technology currently enables these forms of manipulation:
- Add and remove objects within a video.
- Alter background conditions in a video. For example, changing the weather to make a video shot in summer appear as if it was shot in winter.
- Simulate and control a realistic video representation of the lips, facial expressions or body movement of a specific individual. Although the deepfakes discussion generally focuses on faces, similar techniques are being applied to full-body movement, or specific parts of the face.
- Generate a realistic simulation of a specific person’s voice.
- Modify an existing voice with a “voice skin” of a different gender, or of a specific person.
- Create a realistic but totally fake photo of a person who does not exist. The same technique can also be applied less problematically to create fake hamburgers, cats, etc.
- Transfer a realistic face from one person to another, aka a deepfake.
These techniques primarily but not exclusively rely on a form of artificial intelligence known as deep learning and what are called Generative Adversarial Networks, or GANs.
To generate an item of synthetic media content, you begin by collecting images or source video of the person or item you want to fake. A GAN develops the fake — be it video simulations of a real person or face-swaps — by using two networks. One network generates plausible re-creations of the source imagery, while the second network works to detect these forgeries. This detection data is fed back to the network engaged in the creation of forgeries, enabling it to improve.
As of late 2019, many of these techniques — particularly the creation of deepfakes — continue to require significant computational power, an understanding of how to tune your model, and often significant postproduction CGI to improve the final result. However, even with current limitations, humans are already being tricked by simulated media. As an example, research from the FaceForensics++ project showed that people could not reliably detect current forms of lip movement modification, which are used to match someone’s mouth to a new audio track. This means humans are not inherently equipped to detect synthetic media manipulation.
It should also be noted that audio synthesis is advancing faster than expected and becoming commercially available. For example, the Google Cloud Text-to-Speech API enables you to take a piece of text and convert it to audio with a realistic sounding human voice. Recent research has also focused on the possibility of doing text to combined video/audio edits in an interview video.
On top of that, all the technical and commercialization trends indicate that it will continue to become easier and less expensive to make convincing synthetic media. For example, the below image shows how quickly face generation technology has advanced.
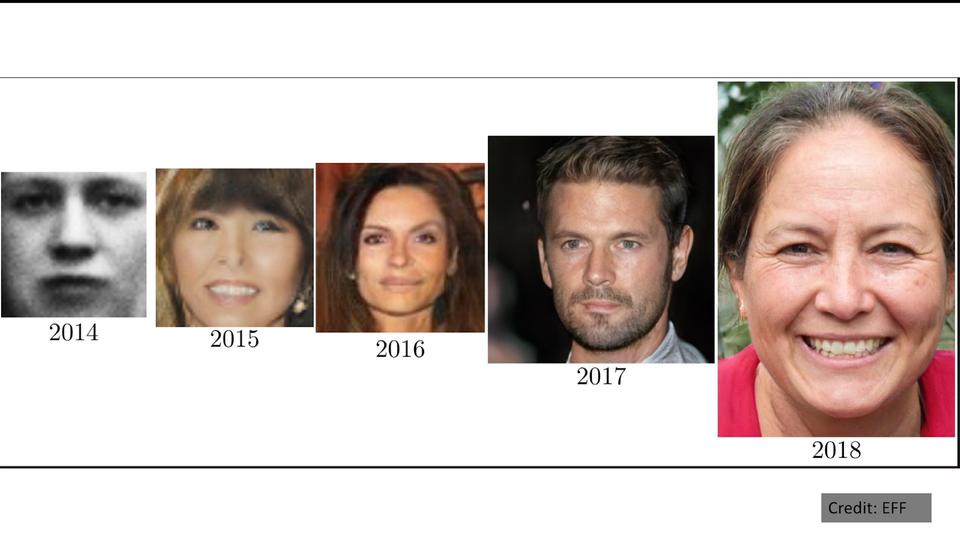
Because of the cat-and-mouse nature of these networks, they improve over time as data on successful forgeries and successful detection is fed through them. This requires strong caution about the effectiveness of detection methods.
The current deepfake and synthetic media landscape
Deepfakes and synthetic media are — as yet — not widespread outside of nonconsensual sexual imagery. DeepTrace Lab’s report on their prevalence as of September 2019 indicates that over 95% of the deepfakes were of this type, either involving celebrities, porn actresses or ordinary people. Additionally, people have started to challenge real content, dismissing it as a deepfake.
In workshops led by WITNESS, we reviewed potential threat vectors with a range of civil society participants, including grassroots media, professional journalists and fact-checkers, as well as misinformation and disinformation researchers and OSINT specialists. They prioritized areas where new forms of manipulation might expand existing threats, introduce new threats, alter existing threats or reinforce other threats. They identified threats to journalists, fact-checkers and open-source investigators, and potential attacks on their processes. They also highlighted the challenges around “it’s a deepfake” as a rhetorical cousin to “it’s fake news.”
In all contexts, they noted the importance of viewing deepfakes in the context of existing approaches to fact-checking and verification. Deepfakes and synthetic media will be integrated into existing conspiracy and disinformation campaigns, drawing on evolving tactics (and responses) in that area, they said.
Here are some specific threats they highlighted:
- Journalists and civic activists will have their reputation and credibility attacked, building on existing forms of online harassment and violence that predominantly target women and minorities. A number of attacks using modified videos have already been made on women journalists, as in the case of the prominent Indian journalist Rana Ayyub.
- Public figures will face nonconsensual sexual imagery and gender-based violence as well as other uses of so-called credible doppelgangers. Local politicians may be particularly vulnerable, as they have plentiful images but less of the institutional structure around them as national-level politicians to help defend against a synthetic media attack. They also often are key sources in news coverage that bubbles up from local to national.
- Appropriation of known brands with falsified in-video editing or other ways in which a news, government, corporate or NGO brand might be falsely attached to a piece of content.
- Attempts to plant manipulated user generated content into the news cycle, combined with other techniques such as source-hacking or sharing manipulated content to journalists at key moments. Typically, the goal is to get journalists to propagate the content.
- Utilization of newsgathering/reporting process weaknesses such as single-camera remote broadcasts (as noted by the Reuters UGC team) and gathering material in hard-to-verify contexts such as war zones or other places.
- As deepfakes become more common and easier to make at volume, they will contribute to a fire hose of falsehood that floods media verification and fact-checking agencies with content they have to verify or debunk. This could overload and distract them.
- Pressure will be on newsgathering and verification organizations to prove that something is true, as well as to prove that something is not falsified. Those in power will have the opportunity to use plausible deniability on content by declaring it is deepfaked.
A starting point for verifying deepfakes
Given the nature of both media forensics and emerging deepfakes technologies, we have to accept that the absence of evidence that something was tampered with will not be conclusive proof that media has not been tampered with.
Journalists and investigators need to establish a mentality of measured skepticism around photos, videos and audio. They must assume that these forms of media will be challenged more frequently as knowledge and fear of deepfakes increases. It’s also essential to develop a strong familiarity with media forensics tools.
With that in mind, an approach to analyzing and verifying deepfakes and synthetic media manipulation should include:
- Reviewing the content for synthetic media-derived telltale glitches or distortions.
- Applying existing video verification and forensics approaches.
- Utilizing emerging new AI-based approaches and emerging forensics approaches when available.
Reviewing for telltale glitches or distortions
This is the least robust approach to identifying deepfakes and other synthetic media modifications, particularly given the evolving nature of the technology. That said, poorly made deepfakes or synthetic content may present some evidence of visible errors. Things to look in a deepfake for include:
- Potential distortions at the forehead/hairline or as a face moves beyond a fixed field of motion.
- Lack of detail on the teeth.
- Excessively smooth skin.
- Absence of blinking.
- A static speaker without any real movement of head or range of expression.
- Glitches when a person turns from facing forward to sideways.
Some of these glitches are currently more likely to be visible on a frame-by-frame analysis, so extracting a series of frames to review individually can help. This will not be the case for the frontal-lateral movement glitches — these are best seen in a sequence, so you should do both approaches.
Applying existing video verification approaches
As with other forms of media manipulation and shallowfakes, such as miscontextualized or edited videos, you should ground your approach in well-established verification practices. Existing OSINT verification practices are still relevant, and a good starting point is the chapters and case studies in the first Handbook dedicated to image and video verification. Since most deepfakes or modifications are currently not fully synthesized but instead rely on making changes in a source video, you can use frames from a video to look for other versions using a reverse image search. You can also check the video to see if the landscape and landmarks are consistent with images of the same location in Google Street View.
Similarly, approaches based on understanding how content is shared, by who, and how may reveal information about whether to trust an image or video. The fundamentals of determining source, date, time and motivation of a piece of content are essential to determining whether it documents a real event or person. (For a basic grounding in this approach, see this First Draft guide.) And as always, it’s essential to contact the person or people featured in the video to seek comment, and to see if they can provide concrete information to support or refute its authenticity.
New tools are also being developed by government, academics, platforms and journalistic innovation labs to assist with the detection of synthetic media, and to broaden the availability of media forensics tools. In most cases, these tools should be viewed as signals to complement your best-practices based verification approach.
Tools such as InVID and Forensically help with both provenance-based image verification and limited forensic analysis.
Free tools in this area of work include:
- FotoForensics: An image forensics tool that includes the capacity for Error Level Analysis to see where elements of an image might have been added.
- Forensically: A suite of tools for detecting cloning, error level analysis, image metadata and a number of other functions.
- InVID: A web browser extension that enables you to fragment videos into frames, perform reverse image search across multiple search engines, enhance and explore frames and images through a magnifying lens, and to apply forensic filters on still images.
- Reveal Image Verification Assistant: A tool with a range of image tampering detection algorithms, plus metadata analysis, GPS geolocation, EXIF thumbnail extraction and integration with reverse image search via Google.
- Ghiro: An open-source online digital forensics tool.
Note that almost all of these are designed for verification of images, not video. This is a weakness in the forensics space, so for videos it is still necessary to extract single images for analysis, which InVID can help with. These tools will be most effective with higher resolution, noncompressed videos that, for example, had video objects removed or added within them. Their utility will decrease the more a video has been compressed, resaved or shared across different social media and video-sharing platforms.
If you’re looking for emerging forensics tools to deal with existing visual forensics issues as well as eventually deepfakes, one option is to look at the tools being shared by academics. One of the leading research centers at the University of Napoli provides online access to their code for, among other areas, detecting camera fingerprints (Noiseprint), detecting image splices (Splicebuster) and detecting copy-move and removal detection in video.
As synthetic media advances, new forms of manual and automatic forensics will be refined and integrated into existing verification tools utilized by journalists and fact-finders as well as potentially into platform-based approaches. It’s important that journalists work to stay up to date on the available tools, while also not becoming overly reliant upon them.
Emerging AI-based and media forensics approaches
As of early 2020, there are no tested, commercially available GAN-based detection tools. But we should anticipate that some will enter the market for journalists either as plug-ins or as tools on platforms in 2020. For a current survey of the state-of-field in media forensics including these tools you should read Luisa Verdoliva’s ‘Media Forensics and Deepfakes: An overview’.
These tools will generally rely on having training data (examples) of GAN-based synthetic media, and then being able to use this to detect other examples that are produced using the same or similar techniques. As an example, forensics programs such as FaceForensics++ generate fakes using existing consumer deepfakes tools and then use these large volumes of fake images as training data for algorithms to perform fake detection. This means they might not be effective on the latest forgery methods and techniques.
These tools will be much more suited to detection of GAN-generated media than current forensic techniques. They will also supplement new forms of media forensics tools that deal better with advances in synthesis. However, they will not be foolproof, given the adversarial nature of how synthetic media evolves. A key takeaway is that any indication of synthesis should be double-checked and corroborated with other verification approaches.
Deepfakes and synthetic media are evolving fast and the technologies are becoming more broadly available, commercialized and easy to use. They need less source content to create a forgery than you might expect. While new technologies for detection emerge and are integrated into platforms and into journalist/OSINT-facing tools, the best way to approach verification is using existing approaches to image/video, and complement these with forensics tools that can detect image manipulation. Trusting the human eye is not a robust strategy!