Sketching With Data
Written by Mona Chalabi and Jonathan Gray
Abstract
An interview with celebrated data journalist Mona Chalabi exploring the development and reception of her practice of sketching as a way of making data relatable, including discussion of data as a means of providing context, visual practices of making things comparable, the role of humour and analogy in her work, data journalism as social commentary, and the importance of communicating the uncertainty of data and the provisionality of analysis.
Keywords: data sketching, data visualization, uncertainty, data publics, data journalism, visual practices
Jonathan Gray (JG): How did you start sketching with data?
Mona Chalabi (MC): When I was working at FiveThirtyEight I felt that they weren’t catering to readers like me. They were catering to a slightly different kind of reader with their complex interactives. During this time I began sketching with data, which I could do while sitting at my desk. As I started to do them I had this realization that they could be quite an effective way to communicate the uncertainty of data projects. They could remind people that a human was responsible for making all of these design decisions. They could be quite democratizing, communicating with data in a way that anyone can do. I used to write this DIY column at The Guardian which took people through every single step of my process. It was fun that as a journalist you could talk people through not only where you found your data, exactly how you processed it and what you did to it, but you could also enable them to replicate it, breaking down the wall between them and you, and hopefully creating new kinds of accessibility, participation and relationships with readers.
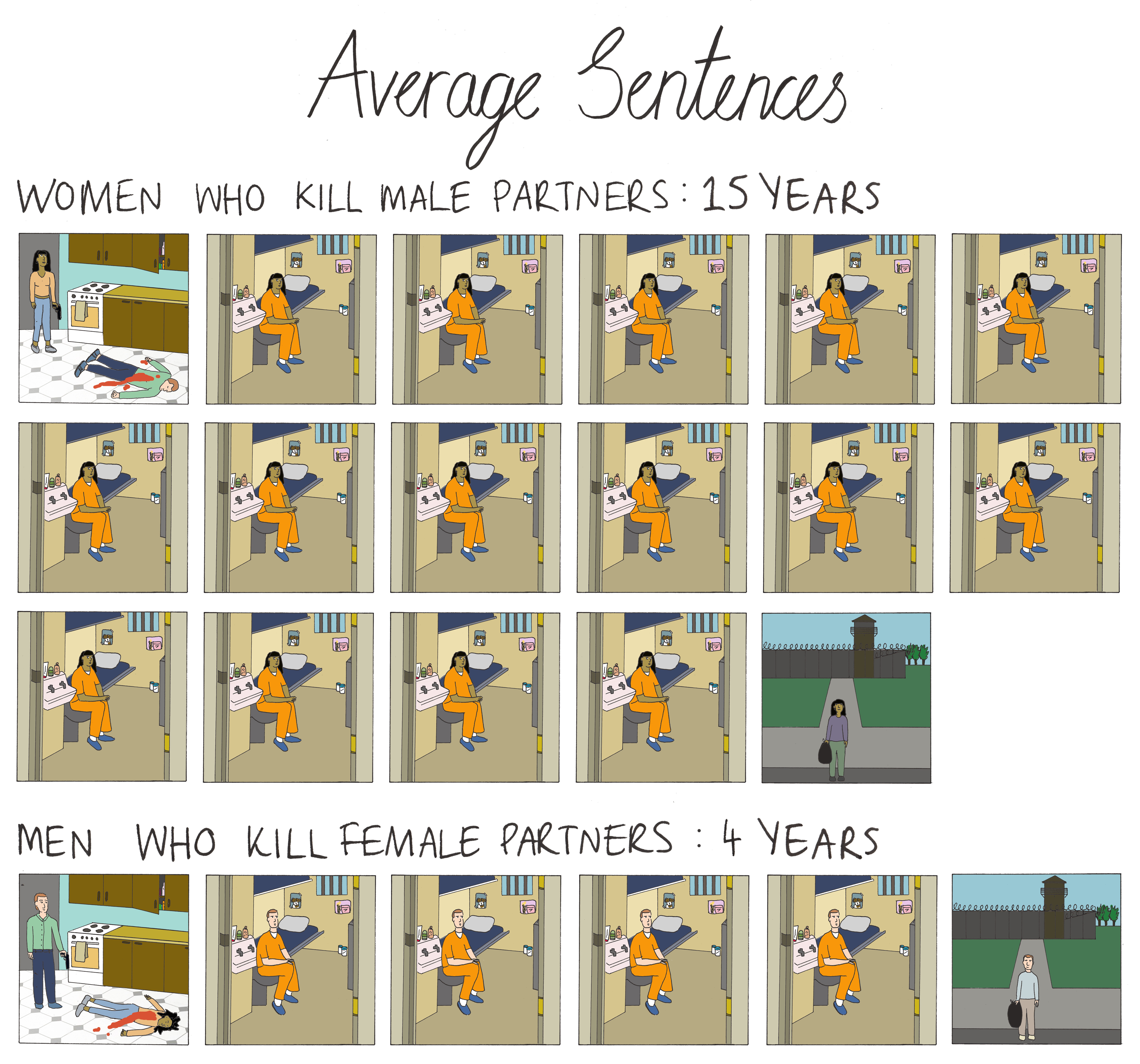
JG: In the book we explore how data journalists do not just have to mirror and reinforce established forms of expertise (e.g., data science and advanced statistical methods), but how they can also promote other kinds of data practices and data cultures. Do you consider your work to be partly about finding other ways of working with and relating to data?
MC: I don’t have really advanced statistical skills. The way that I often start analyzing data is through relatively simple calculations that other people can replicate. In a way this makes the data that I’m using much more reliable. At a certain point with other more advanced statistical approaches you present readers with an ultimatum: Either you trust the journalist’s analysis or you don’t. This is different to the proposition of trusting government statistics and basic multiplication or not trusting them. There is a certain benefit to doing things with simple calculations. This is a big part of what I do and my approach.
Data can be used as an opportunity to do two different things: To “zoom in” or “zoom out.” On the one hand, my responsibility as a data journalist is to zoom out from that one specific incident and give readers context using data. For example, say there is an incident or an attack, we might show them how these attacks happen, where they happen, whether their prevalence increases over time and whether there are people who are more targeted than others. That is an opportunity for readers to understand broader trends, which can be really informative for them. Maybe it helps them to not freak out, or to duly freak out in response to the news.
On the other hand, we can do the complete opposite and zoom in. Let’s say that the BLS [US Bureau of Labor Statistics] publishes unemployment data and that most other news outlets just publish the unemployment rate. We as data journalists are able to zoom in: We can say to readers, here is the national employment rate but also this is what it looks like for women, this is what it looks like for men, this is what it looks like for different age groups, here is what it looks like for different racial and ethnic groups. So it allows readers to explore the data more closely.
My work alternates between these two modes. I think one of my biggest critiques of outlets like FiveThirtyEight is that the work can sometimes be about intellectual bravado: “Here’s what we can do.” I’m not into that. My purpose is to serve readers and in particular the broadest community of readers, not just White men who identify as geeks. FiveThirtyEight readers call themselves geeks and FiveThirtyEight journalists call themselves that. But that is not why I got into journalism.
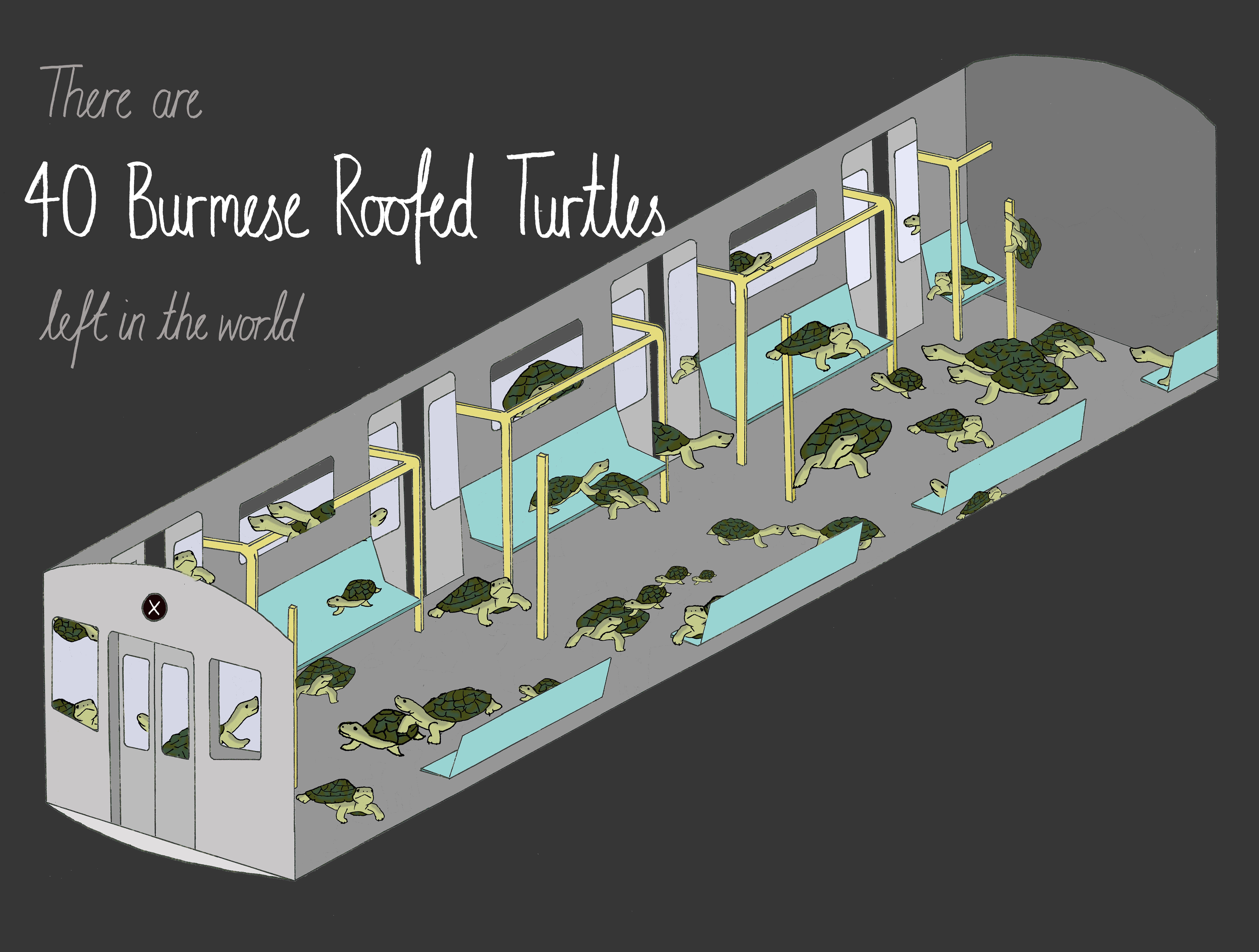
JG: To take one recent example of your work, could you tell us a bit more about the “Endangered Species on a Train” piece published in The Guardian (Figure 25.2)? How did you get into this topic, how did the project arise and how did you approach it?
MC: It was actually quite strange. It was not really inspired by the news; it was more about my personal practice of doing these illustrations and wanting to do something a bit more ambitious. Part of the reason why I started doing these illustrations is they are also really efficient: They can have such a fast turnaround, and can be made in a matter of hours if need be. I wanted to create something bigger that would take a bit more time. I started with a much bigger topic that people already feel familiar with—endangered species—but for which the existing visual language is perhaps a bit uninspiring. I took data from the International Union for Conservation of Nature (IUCN) “Red List.”1 For a lot of those numbers on endangered species they gave a range, and I chose a midpoint for each of them.
Stepping back, you could look at my illustrations as charts. The only thing that makes them charts is scale. Every illustration that I post has a sense of scale and that is what every single chart does. One of the problems with scale is that different countries and places use different scales, for example, millimetres in the United Kingdom and inches in the United States. Scales mean different things to different people. A lot of data journalists lose sight of this. What does “1 million” mean to someone? What does “1” mean to someone? All of this depends on context. When numbers are low it can be easier to get your head around this: You know what 27 means. But what does that mean?
Part of the beauty of data visualization is that it can make things feel more visceral. Another illustration that I was pretty proud of and that did really well was one where I compared the average parking space to the average solitary confinement cell (Figure 25.3). This is like a common practice for dealing with numbers in journalism: You don’t say “bankers in London earn this much,” you say “bankers in London earn 7,000 times what a social worker earns.” All of those analogies really help people.
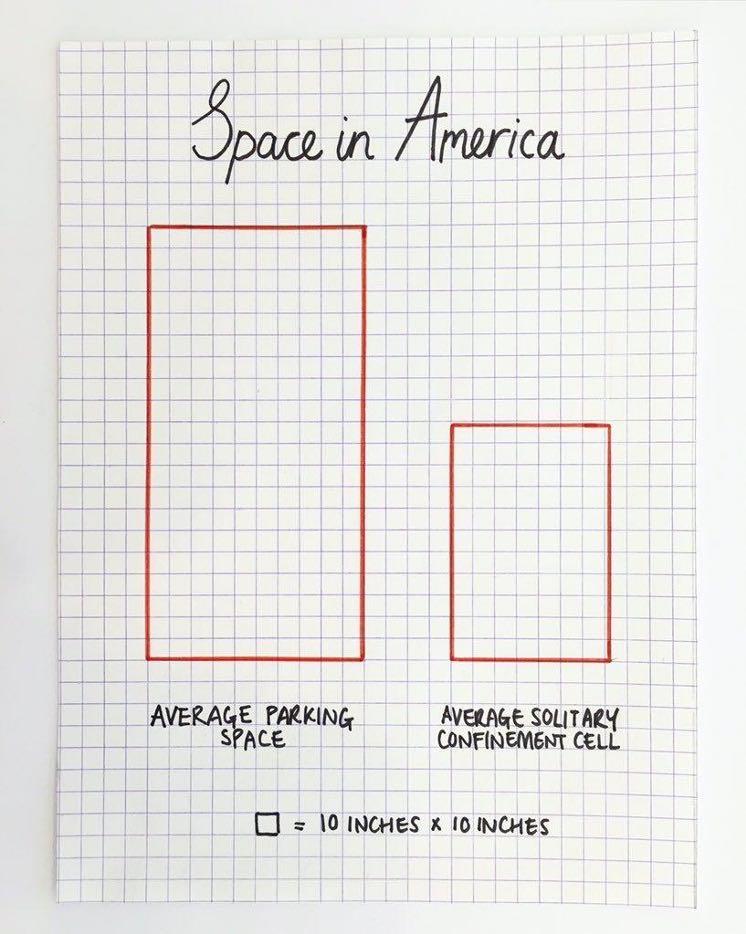
JG: It seems that part of your practice is also to do with juxtaposition of different elements (e.g., the familiar and the disturbing). Is there also a curatorial element here?
MC: Humour also plays an important role in my work. Not that the pieces are funny, but there is often something wry in the style. The best comedy is basically saying “this is fucked up.” There is always some kind of social commentary. If you can inject data journalism with a little bit of that it can be really powerful.
JG: Returning to the “Endangered Species” example as a case of making numbers relatable through humour and the use of different visual spaces of comparison, did you start with the carriage (as opposed to the chart)?
MC: First I drew the carriage, and then I drew about seven or eight of each animal. I used Photoshop to separate out the layers, to colour them and to count them. To make sure I got it correct each animal is a different layer. My first idea was to draw endangered species in different things which are all universally relatable.
The New York Subway is not perfect (is it bigger or smaller than the London Tube?), but it is enough to give you a sense of scale. I started with a spreadsheet of different possibilities combining endangered species and relatable spaces. I was thinking of showing a shark in a swimming pool. But with all of the different spaces it felt a bit difficult to get your head around and once I started drawing them I realized it was going to be a really lengthy process. Rather than drawing them all in different places I would show them all in the same one, which also works better.
It is not really perfect: To fit all of the rhinos in the scale is a little bit questionable I would say (a lot of them would need to be babies rather than adults!). But it makes you feel something about the numbers. And it is also transparent about its shortcomings.
When you look at a chart that FiveThirtyEight created, how are you, especially as a non-expert, supposed to remotely understand to what extent it is accurate? Readers are just given an ultimatum: Trust us or don’t. When readers look at the illustrations of the endangered species they can look at the rhinos and think, “It is a little bit off but I get it.” They have access to that critique in a way that they don’t with computer generated graphics.
JG: Earlier you mentioned that you hoped your work could democratize how people engage with data. Could you say a bit more about this?
MC: Without this ability for readers to participate in making sense with data and forming their own judgements, how are journalists any better than politicians? You have right-wing papers and left-wing papers just saying: “You either trust us or you don’t.”
But we’re supposed to be empowering people to make informed decisions in their everyday lives. Empowering people is not just about saying, “These are the facts, now clearly you’re supposed to go and do this.” It is saying, “These are the facts; here is how we got here.” It is not just journalism: I think there is a lot of work to be done in medicine as well. I’d like to do more work around how to change medical packaging. Rather than boxes saying, “Here’s what you need to do,” if you’re going to be a really good doctor you should be able to say to the patient, “These are the risks for this medicine. These are the risks of not taking it. These are the risks of this other course of medicine. These are the risks of not taking it,” so people can make decisions for themselves as no two people are alike.
I think good data visualizations should communicate uncertainty.2 Uncertainty is part of that whole story of making an informed decision in your life. So few data journalists take the time to communicate uncertainty. So few data journalists take the time to reach out to communities that aren’t geeks. Just because you don’t have these particular vocabularies of statistical or computational skills does that mean that you are not smart, that you are not entitled to understand this information? Of course not. And yet some data journalists refer to so many of these terms in this off-hand way, like, “I’m not going to bother explaining this every time. You either get it or you don’t.” It is stupid. My approach to data journalism is based on the idea that you don’t necessarily need specific vocabularies or expertise to be smart.
JG: Is there also an element of people participating in deciding what matters?
MC: Part of the reason I started the “Dear Mona” advice column was so that people could send me questions. People are constantly sending DMs on Instagram about things which matter to them, and there are many things that I wouldn’t necessarily have thought of at all. There are some routes that I don’t want to go down, like looking at the relation between mental health and gun control, which can stigmatize people with mental health issues and open a whole can of worms. But if I get many DMs from people who want to know about this then you wonder whether you should not just sidestep the nuance because it is complicated but should instead try to tackle it head on. So I’m constantly looking to readers to tell me what matters to them. I don’t think that this is an abdication of journalistic responsibility. It is part of the democratic role of journalism and people seeing that they have a stake in the final product in every single way: In the process of creating it, in understanding it, and it is not this thing which is just given to them in a “take it or leave it” kind of way.
JG: Could you tell us a bit about the responses to your work? Have there been any unexpected or notable responses?
MC: I get all kinds of different responses to my work. Some people focus on the subject matter. So any time I do something on wage gaps, for example, I get lots of White men that are, like, “No, Black women only earn less because they work less,” and you have to engage with them about how the illustrations are based on “like for like” comparisons between full-time workers, and if there are differences in the levels they are at (e.g., senior management), that is also part of the problem. I’m always keen to focus on the critique first.
But overall I get much more support than criticism. Sometimes people respond to critiques in comments even before I get to them. People whose lives are represented in the illustrations sometimes intervene to say, “No, my personal experience bears this out.” People sometimes want to see extra data. Lots of students write to say that they really want to do this (interestingly I get more female students writing to me than men). A lot of NGOs and charities write to me as they want to feel something about their data rather than thinking something about their data, and sometimes my work manages to do that. One of my pieces was cited in a US bill.
My work has been viewed and shared by a lot of people on social media who are not necessarily into data journalism per se, which is getting it in front of a new audience. Bernie Sanders shared my gun violence illustration, Miley Cyrus shared one, as did Iman, the model, and Shaun King, the civil rights activist. These are not people I know and not necessarily people who follow my work, but they see other people sharing it and it somehow ends up on their radar. It is amazing to see people engaging with it. Once someone prominent shares it, it can take on a life of its own sometimes.
Examples of the works referred to in this chapter can be found on the web at monachalabi.com and on Instagram at @monachalabi.
Footnotes
1. www.iucnredlist.org
2. Editors: See also Anderson’s chapter in this book, as well as his Apostles of Certainty: Data Journalism and the Politics of Doubt (Oxford University Press, 2018).