Building Your Own Data Set: Documenting Knife Crime in the United Kingdom
Written by Caelainn Barr
Abstract
Building data sets for investigations and powerful storytelling.
Keywords: data journalism, crime, accountability, race, United Kingdom, databases
In early 2017 two colleagues, Gary Younge and Damien Gayle, approached me in The Guardian newsroom. They wanted to examine knife crime in the United Kingdom. While there was no shortage of write-ups detailing the deaths of victims of knife crime, follow-ups on the pursuit of suspects, and reports on the trials and convictions of the perpetrators, no one had looked at all the homicides as a whole.
My first question was, how many children and teenagers had been killed by knives in recent years?
It seemed a straightforward query but once I set out to find the data it soon became apparent—no one could tell me. The data existed, somewhere, but it wasn’t in the public domain. At this stage I had two options, give up or make a data set from scratch based on what I could access, build and verify myself. I decided to build my own data set.
Why Build Your Own Data Set?
Data journalism needn’t be solely based on existing data sets. In fact there is a great case for making your own data. There is a wealth of information in data that is not routinely published or in some cases not even collected.
In building your own data set you create a unique set of information, a one-off source, with which to explore your story. The data and subsequent stories are likely to be exclusive and it can give you a competitive edge to find stories other reporters simply can’t. Unique data sets can also help you identify what trends experts and policy makers haven’t been able to spot.
Data is a source of information in journalism. The basis for using data in journalism is structured thinking. In order to use data to its full potential, at the outset of a project the journalist needs to think structurally: What is the story I want to be able to tell and what do I need to be able to tell it?
The key to successfully building a data set for your story is to have a structured approach to your story and query every source of data with a journalistic sense of curiosity.
Building your own data set encompasses a lot of the vital skills of data journalism—thinking structurally, planned storytelling and finding data in creative ways. It also has a relatively low barrier to entry, as it can be done with or without programming skills. If you can type into a spreadsheet and sort a table, you’re on your way to building the basic skills of data journalism.
That’s not to say data journalism is straightforward. Solid and thorough data projects can be very complex and time-consuming work, but armed with a few key skills you can develop a strong foundation in using data for storytelling.
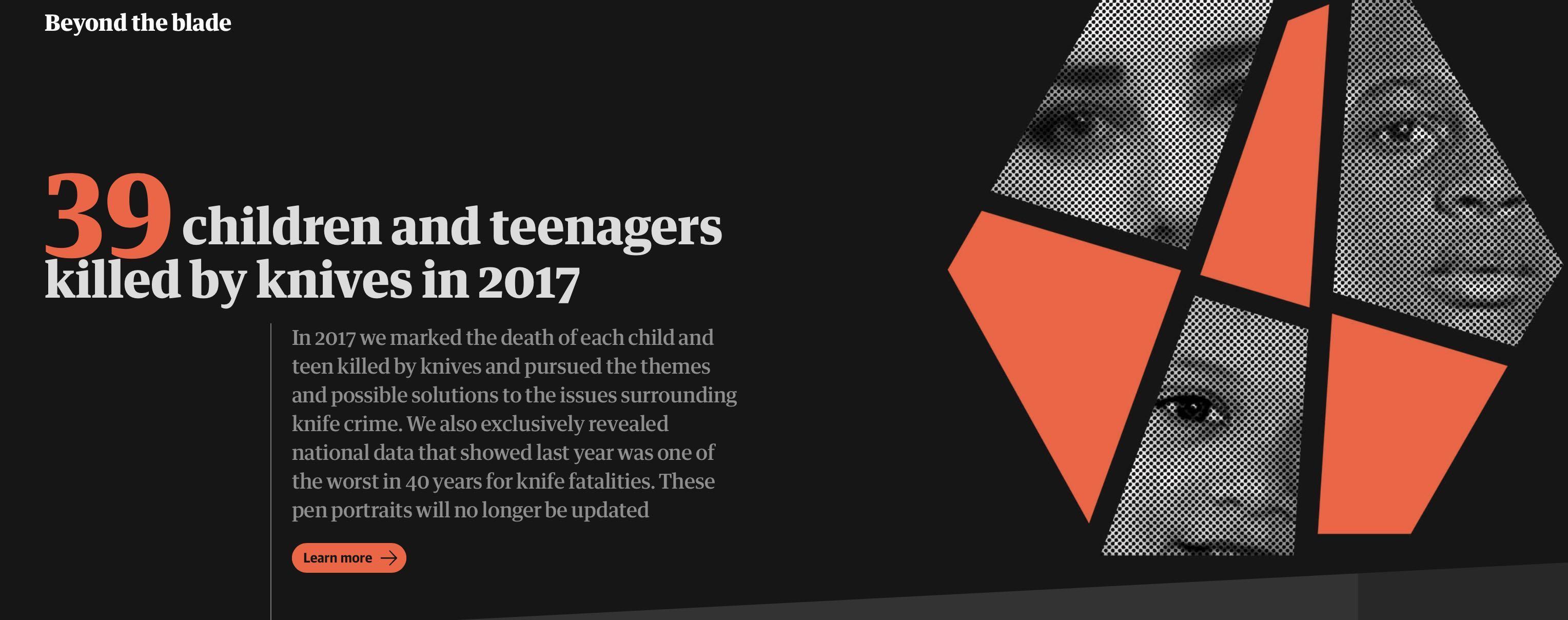
Building Your Own Data Set Step by Step
Plan what is required. The first step to making or gathering data for your analysis is assessing what is required and if it can be obtained. At the outset of any project it’s worth making a story memo which sketches out what you expect the story will attempt to tell, where you think the data is, how long it will take to find it and where the potential pitfalls are. The memo will help you assess how long the work will take and if the outcome is worth the effort. It can also serve as a something to come back to when you’re in the midst of the work at a later stage.
Think of the top line. At the outset of a data-driven story where the data does not exist you should ask what the top line of the story is. It’s essential to know what the data should contain as this sets the parameters for what questions you can ask of the data. This is essential as the data will only ever answer questions based on what it contains. Therefore, to make a data set that will fulfil your needs, be very clear about what you want to be able to explore and what information you need to explore it.
Where might the data be held? The next step is to think through where the data may be held in any shape or form. One way to do this is to retrace your steps. How do you know there is a potential story here? Where did the idea come from and is there a potential data source behind it?
Research will also help you clarify what exists, so comb through all of the sources of information that refer to the issue of interest and talk to academics, researchers and statisticians who gather or work with the data. This will help you identify shortcomings and possible pitfalls in using the data. It should also spark ideas about other sources and ways of getting the data. All of this preparation before you start to build your data set will be invaluable if you need to work with difficult government agencies or decide to take another approach to gathering the data.
Ethical concerns. In planning and sourcing any story we need to weigh up the ethical concerns and working with data is no different. When building a data set we need to consider if the source and method we’re using to collect the information is the most accurate and complete possible.
This is also the case with analysis—examine the information from multiple angles and don’t torture the data to get it to say something that is not a fair reflection of the reality. In presenting the story be prepared to be transparent about the sourcing, analysis and limitations of the data. All of these considerations will help build a stronger story and develop trust with the reader.
Get the data. Once a potential source has been identified, the next step is to get the data. This may be done manually through data entry into a spreadsheet, transforming information locked in PDFs into structured data you can analyze, procuring documents through a human source or the Freedom of Information Act (FOIA), programming to scrape data from documents or web pages or automating data capture through an application programming interface (API).
Be kind to yourself! Don’t sacrifice simplicity for the sake of it. Seek to find the most straightforward way of getting the information into a data set you can analyze. If possible, make your work process replicable, as this will help you check your work and add to the data set at a later stage, if needed.
In obtaining the data refer back to your story outline and ask, will the data allow me to fully explore this topic? Does it contain the information that might lead to the top lines I’m interested in?
Structure. The key difference between information contained in a stack of text-based paper documents and a data set is structure. Structure and repetition are essential to building a clean data set ready for analysis.
The first step is to familiarize yourself with the information. Ask yourself what the material contains—what will it allow you to say? What won’t you be able to say with the data? Is there another data set you might want to combine the information with? Can you take steps in building this data set which will allow you to combine it with others?
Think of what the data set should look like at the end of the process. Consider the columns or variables you would want to be able to analyze. Look for inspiration in the methodology and structure underlying other similar data sets.
Cast the net wide to begin with, taking account of all the data you could gather and then pare it back by assessing what you need for the story and how long it will take to get it. Make sure the data you collect will compare like with like. Choose a format and stick to it—this will save you time in the end! Also consider the dimensions of the data set you’re creating. Times and dates will allow you to analyze the information over time; geographic information will allow you to possibly plot the data to look for spatial trends.
Keep track of your work and check as you go. Keep notes of the sources you have used to create your data set and always keep a copy of the original documents and data sets. Write up a methodology and a data dictionary to keep track of your sources, how the data has been processed and what each column contains. This will help flag questions and shake out any potential errors as you gather and start to analyze the data.
Assume nothing and check all your findings with further reporting. Don’t hold off talking to experts and statisticians to sense–check your approach and findings. The onus to bulletproof your work is even greater when you have collated the data, so take every step to ensure the data, analysis and write-up are correct.
Case Study: Beyond the Blade
At the beginning of 2017 the data projects team alongside Gary Younge, Damian Gayle and The Guardian’s community journalism team set out to document the death of every child and teenager killed by a knife in the United Kingdom. In order to truly understand the issue and explore the key themes around knife crime the team needed data. We wanted to know—who are the young people dying in the United Kingdom as a result of stabbings? Are they young children or teenagers? What about sex and ethnicity? Where and when are these young people being killed?
After talking to statisticians, police officers and criminologists it became clear that the data existed but it was not public. Trying to piece together an answer to the question would consume much of my work over the next year.
The data I needed was held by the Home Office in a data set called the Homicide Index. The figures were reported to the Home Office by police forces in England and Wales. I had two potential routes to get the information—send a freedom of information request to the Home Office or send requests to every police force. To cover all eventualities, I did both. This would provide us with the historical figures back to 1977.
In order to track deaths in the current year we needed to begin counting the deaths as they happened. As there was no public or centrally collated data we decided to keep track of the information ourselves, through police reports, news clippings, Google Alerts, Facebook and Twitter.
We brainstormed what we wanted to know—name, age and date of the incident were all things we definitely wanted to record. But other aspects of the circumstances of the deaths were not so obvious. We discussed what we thought we already knew about knife crime—it was mostly male with a disproportionate number of Black male victims.
To check our assumptions we added columns for sex and ethnicity. We verified all the figures by checking the details with police forces across the United Kingdom. In some instances this revealed cases we hadn’t picked up and allowed us to cross-check our findings before reporting.
After a number of rejected FOI requests and lengthy delays the data was eventually released by the Home Office. It gave the age, ethnicity and sex of all people killed by knives by police force area for almost 40 years. This, combined with our current data set, allowed us to look at who was being killed and the trend over time.
The data revealed knife crime had killed 39 children and teenagers in England and Wales in 2017, making it one of the worst years for deaths of young people in nearly a decade. The figures raised concerns about a hidden public health crisis amid years of police cuts.
The figures also challenged commonly held assumptions about who knife crime affects. The data showed in England and Wales in the 10 years to 2015, one third of the victims were Black. However, outside the capital, stabbing deaths among young people were not mostly among Black boys, as in the same period less than one in five victims outside London were Black.
Although knife crime was a much-debated topic, the figures were not readily available to politicians and policy makers, prompting questions about how effective policy could be created when the basic details of who knife crime affects were not accessible.
The data provided the basis of our award-winning project which reframed the debate on knife crime. The project would not have been possible without building our own data set.