2020年Sigma数据新闻奖得奖名单
Sigma数据新闻奖是一个全新的奖项。该奖的设立不仅是为了表彰全球最优秀的数据新闻作品,更希望为数据新闻工作者们赋能,提升并引领他们在这一领域持续前进。第一届Sigma数据新闻奖的报名已于2020年2月5日结束,收到来自全世界66个国家和地区的共计510件作品报名。
由24位国际业界专家组成的评审团(详细名单于网页下方)仔细审阅了全部报名作品,并从6个组别里挑选出格外优秀10份得奖作品以及两份荣誉奖。这些作品体现了全球最出色的数据新闻报道,其中包括来自中国的南华早报(最佳数据可视化报道荣誉奖)。得奖团队的成员将受邀出席今年4月在意大利佩鲁贾举办的2020年国际新闻节,并在活动上向来自全球各国的数据新闻同行呈现他们的作品,同时参与相关的活动环节。
得奖名单:2019年最优秀的数据新闻作品
以下是2020年Sigma数据新闻奖的得奖作品名单
最佳数据驱动报道(大型新闻机构)
得奖作品: The Troika Laundromat
机构: OCCRP(罗马尼亚), The Guardian(英国), Süddeutsche Zeitung(德国), Newstapa(韩国), El Periodico(西班牙), Global Witness以及另外17家伙伴媒体。
作者: Coordinators: Paul Radu, Sarunas Cerniauskas. Reporters: Olesya Shmagun, Dmitry Velikovsky, Alesya Marohovskaya, Jason Shea, Jonny Wrate, Atanas Tchobanov, Ani Hovhannisyan, Irina Dolinina, Roman Shleynov, Alisa Kustikova, Edik Baghdasaryan, Vlad Lavrov
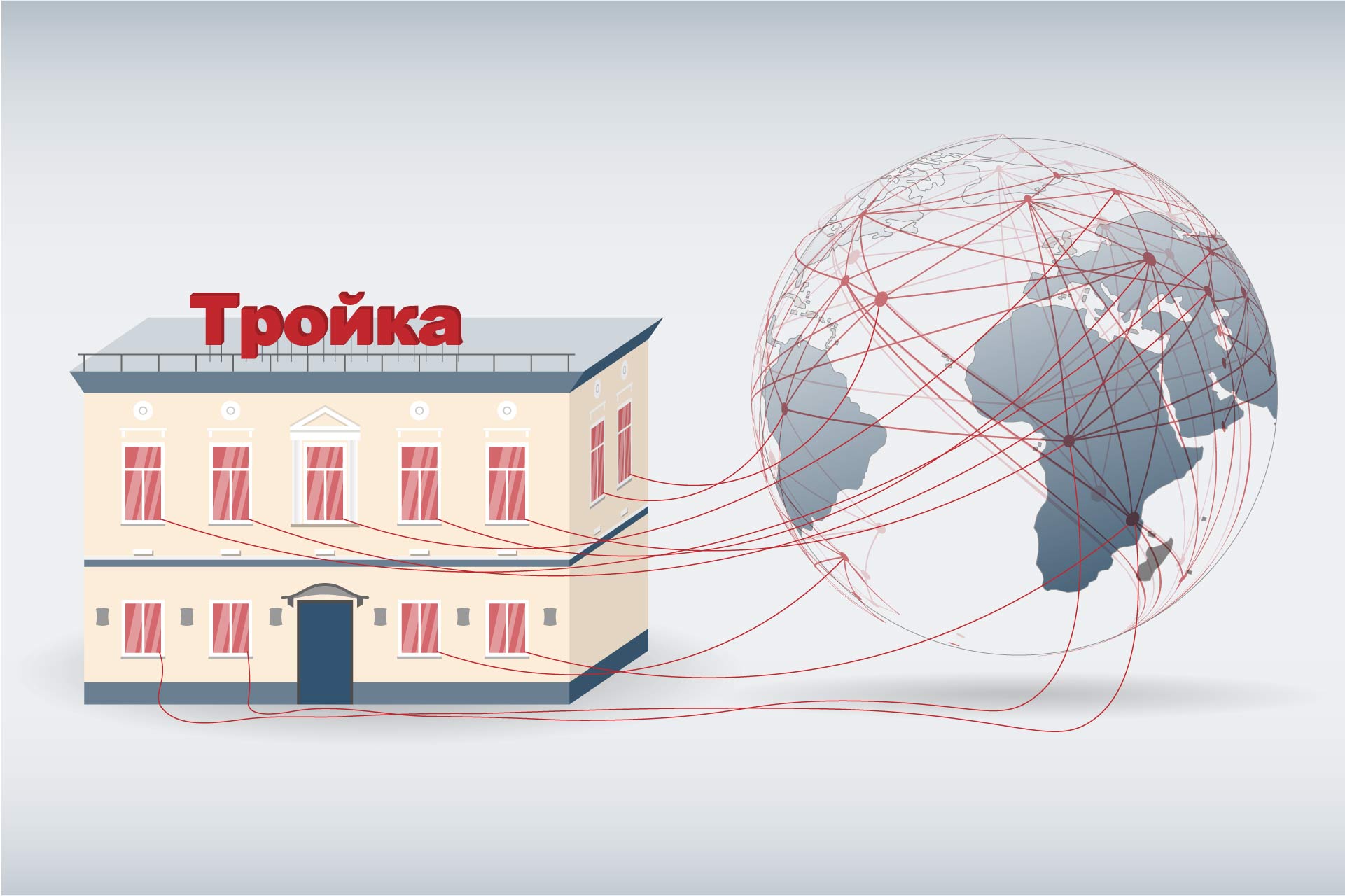
评审团评语: In a field of strong entries, the substantial effort, investment and not inconsiderable risk in piecing this story together, were some of the factors appreciated by the jury in selecting the Troika Laundromat, by the Organized Crime and Corruption Reporting Project (OCCRP) as the winner in this category. This far-reaching investigation touched almost 3000 companies across 15 countries and as many banks, unveiling more than €26 billion in transfers tracked for 7 year period (2006-2013), with the main purpose of ‘channeling money out of Russia.’ The security and scrutiny undertaken for a project of this size is evident with real consequences for political leaders. The showcasing of detail in networks, locations and personalities embellished an already strong entry. This project in places read part thriller, part blockbuster, part spy movie. Do yourself a favour and dive in.
机构类别: 大型机构
发表日期: 4 Mar 2019
作品简介: We exposed a complex financial system that allowed Russian oligarchs and politicians in the highest echelons of power to secretly invest their ill-gotten millions, launder money, evade taxes, acquire shares in state-owned companies, buy real estate in Russia and abroad, and much more. The Troika Laundromat was designed to hide the people behind these transactions and was discovered by OCCRP and its partners through careful data analysis and thorough investigative work in one of the largest releases of banking information, involving some 1.3 million leaked transactions from 238,000 companies. A video explainer: https://youtu.be/uteIMGxor0o
作品效果与影响力: First published in March 2019, with stories being added on an ongoing basis, the impact of of the Troika Laundromat was immediate and widespread. Raiffeisen, Citibank, Danske Bank, Nordea Bank, Swedbank, Credit Agricole, and Deutsche Bank were all seemingly implicated, and two banks -- Raiffeisen in Austria and Nordea in Finland -- deeply involved in the Laundromat saw their shares tumble. Twenty-one members of the European Parliament demanded sanctions against bankers whose financial institutions were involved in the money-laundering scheme. They also called for an "EU-wide anti-money laundering supervisory authority." At the same time, the Parliamentary Assembly of the Council of Europe (PACE) called for swift and substantial action to strengthen anti-money laundering provisions and improve international cooperation in the fight against laundromats. The investigation triggered a major political crisis for the president of Cyprus as we revealed that a law firm he established and co-owned, and in which he was a partner at the time, was arranging business deals linked to a friend of Russian President Vladimir Putin, the infamous Magnitsky scandal, and a network of companies used in various financial crimes. It also ignited investigations into some of Russia's most powerful politicians including an investigation in Spain into the property owned by the family of Sergei Chemezov – the president of the main State owned technology conglomerate in Russia, Rostec Corporation, and a former partner of Vladimir Putin in their KGB heydays in Dresden, East Germany. More recently, Sweden's SEB bank was revealed to be caught up in the Laundromat when leaked data raised questions about its dealings with non-resident clients. Overall, the Troika Laundromat put the European banking system under increased scrutiny and is currently brought up in the European institutions as a main reason to clean up the European financial system.
技术/科技: We received the data in various formats, including PDFs, Excel files and CSVs. We built our own virtual banking database, code-named SPINCYCLE. After grouping the source data by the given columns and format, we were left with 68 different structures. For each structure, we built individual Python parsing scripts that would feed data into the SPINCYCLE database. In the database, we organized the transactions so the data would link up. We used a proprietary IBAN API to pull details on banks that were missing in the data. For monetary values, we performed currency conversion at the time of the transaction, so we linked SPINCYCLE to an on-line table of historic exchange rates. We also tagged the accounts for which we had received information so that we could look at the overall flow of funds from the money laundering system. The neural net was trained using data from company registries and the Panama Papers, and it helped us to pick the names of 22,000 individuals from the 250,000 parties involved in the money laundering system. To make the data available to our members, we provided a web-based SQL interface. Later, we added a full-text search index based on ElasticSearch, which could be searched using Kibana as an interface. We also used Aleph, our home-grown open source data analysis engine. On the landing page we aimed to present an overview of the whole network with a chord diagram and a dashboard that sets the model for the whole exploration: a big graphic on top followed by a dashboard with main key points. For the data visualization section we used client side Quasar Framework over Vue.js and D3.js for the graphs, all designed in Adobe Creative Suite. The collaboration took place via the OCCRP secured wiki and Signal.
作品最难完成的部分: The Troika Laundromat was born out of data work done on a large set of very dry banking transactions. We had to look for patterns in order to identify and isolate transactions that stemmed from what we later defined as the Troika Luandormat (TL). You can think of the TL as a TOR-like service meant to anonymize banking transactions. We had to look for the error, for the bad link, in order to identify who was the organizer and who were the users of the system. We finally found out through careful data analysis that the bankers putting this together made a small but fatal mistake: they used only three of their offshore companies to make payments to formation agents in order to set up dozens of other offshore companies that were themselves involved in transacting billions of dollars. These payments which were only in the hundreds of dollars each were of course lost in a sea of millions much larger transactions so we had to find them and realize that they were part of a pattern. The whole Troika Laundromat came in focus after this realization. Another hard part with this particular project was the security of the team's members. The people we reported on were very powerful in their own countries and across borders and we had to insure the communication with reporters in Russia, Armenia and other places was always done via secure channels. Last but not least the factchecking had to be done across borders and across documents and audio in many languages so this took quite a bit of time and effort to make sure we had things right.
作品值得学习的地方: We learned, once again, that it is the combination between deep data analysis and the traditional footwork that makes good investigative journalism. It is the ability to zoom in and out between the data and the reality in the field that can find you the hidden gems. We had a data scientist working with the investigative teams and this cooperation proved to be a recipe for success. We also insured that journalists had multiple entry points, trimmed down to their technical abilities, with the data. The secured wiki where we shared our findings had a section where we described in detail how the information can be accessed through different systems. This was also a place where advanced journalists shared their ready made formulas so that others could apply them on top of their data of interest. We have also learnt in previous projects and applied it here that the data scientist and our data journalists need to be available via Signal to the new arrivals in the collaborative team and be ready to explain how the systems work, what we already found in the data etc. This made their integration much easier and improved efficiency as the new journalists in the project did not have to start from scratch. Another important lesson that we drew is that it is not just cooperation across countries and between very smart reporters that makes a good project but cooperation across leaks can give you a fuller picture. In addition to the new leaked files, reporters on the Troika Laundromat used documents from previous ICIJ investigations, including Offshore Leaks, Panama Papers and Paradise Papers. It's crucial that at some point in time we unify all these datasets as there are many untold stories in the current gaps between them.
作品链接:
- https://www.occrp.org/en/troikalaundromat/vast-offshore-network-moved-billions-with-help-from-major-russian-bank
- https://cdn.occrp.org/projects/kremlins-laundromat/#/overview/companies
- https://www.occrp.org/en/troikalaundromat/laundromat-money-leads-to-spanish-paradise
- https://www.occrp.org/en/troikalaundromat/death-in-vienna
- https://www.occrp.org/en/troikalaundromat/prince-in-wonderland
最佳数据驱动报道(大型新闻机构)
荣誉奖:Copy, Paste, Legislate
机构: USA TODAY, The Center for Public Integrity, The Arizona Republic
国家: 美国
作者: 团队作品
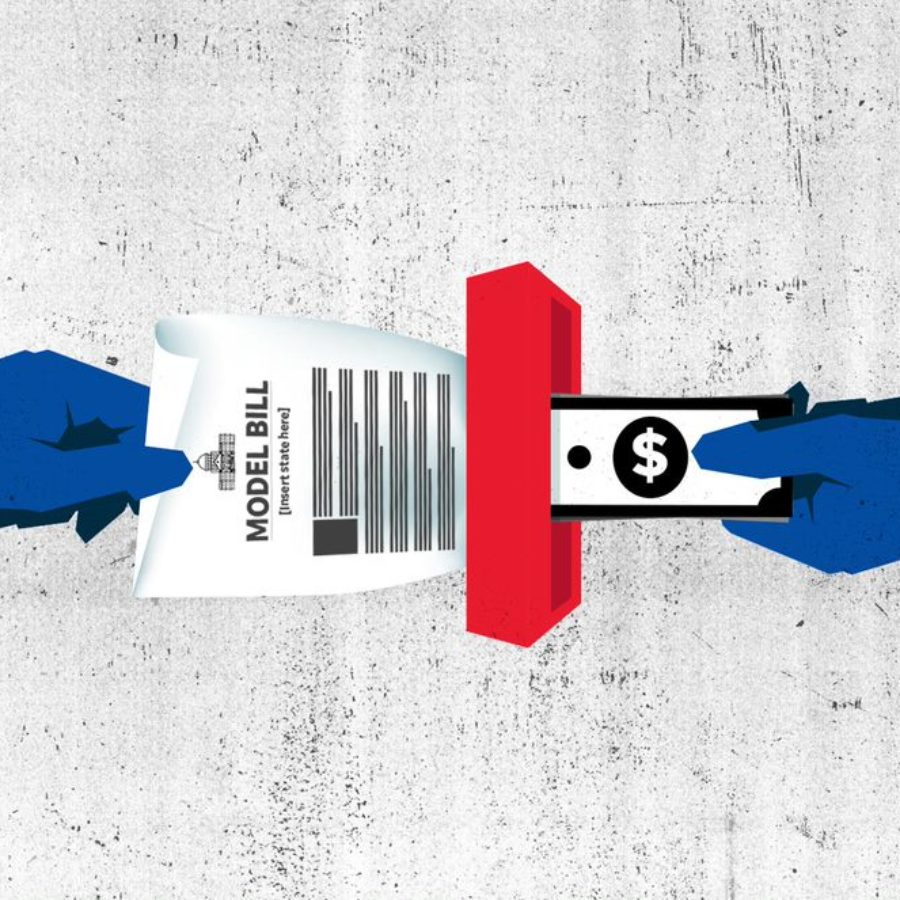
评审团评语: The Arizona Republic, USA Today Network and the Center for Public Integrity analyzed the language of proposed legislation in all 50 states, revealing 10,000 nearly identical bills. Their sophisticated methods revealed the extent of corporate lobbyists and interest group influence on the day-to-day lives of ordinary people, all conducted behind closed doors in statehouses around the U.S.
机构类别: 大型机构
发表日期: 6 Feb 2019
作品简介: Copy, Paste, Legislate marks the first time a news organization detailed how deeply legislation enacted into law at the state level is influenced by special interests in a practice known as "model legislation." The series explained how model legislation was used by auto dealers to sell recalled used cars; by anti-abortion advocates to push further restrictions; by far-right groups to advocate for what some called government-sanctioned Islamophobia to moves by the Catholic Church to limit their exposure to past child abuse claims. (Published February 6, April 3, May 23, June 19, July 17 and October 2, 2019)
作品效果与影响力: People in various states called for legislation to require more transparency about the origin of bill language. Legislators found themselves compelled to defend their sponsorship of model bills. A public-facing model legislation tracker tool launched in November 2019, allowed journalists and the public to: --Identify recent model legislation introduced nationally --Identify recent model legislation introduced in their state --Perform a national search for model legislation mentioning specific keywords or topics --Upload a document they have to instantly identify if any language in their document matches any state legislation introduced since 2010 --Look up a specific bill by number to see all other bills matching it --Look up individual legislators and see all bills sponsored by them that contain model language As part of the project, local newsrooms were able to identify and interview major sponsors of model legislation and identified key issues that resonated in their state. Those stories explored the reach of model legislation and its surprising impact on policies across the nation. The combined national and local reporting revealed: --More than 10,000 bills introduced in statehouses nationwide were almost entirely copied from bills written by special interests --The largest block of special interest bills — more than 4,000 — were aimed at achieving conservative goals --More than 2,100 of the bills were signed into law --The model bills amount to the nation’s largest unreported special interest campaign, touching nearly every area of public policy --Models were drafted with deceptive titles to disguise their true intent, including “transparency” bills that made it harder to sue corporations --Because copycat bills have become so intertwined with the lawmaking process, the nation’s most prolific sponsor of model legislation claimed that he had no idea he had authored 72 bills originally written by outside interests.
技术/科技: No news organization had attempted to put a number on how many of the bills debated in statehouses are substantially copied from those pushed by special interests. We obtained metadata on more than 1 million pieces of legislation from all 50 states for the years 2010 through 2018 from a third-party vendor, Legiscan. We also scraped bill text associated with these bills from the websites of state legislatures. In addition, we pieced together a database of 2,000 pieces of model legislation by getting data from sources, downloading data from advocacy organizations and searching for models ourselves. This was done either by identifying known models and trying to find the source or finding organizations that have pushed model bills and searching for each of the models for which they have advocated. We then compared the two data sets, which proved to be complicated. The team developed an algorithm that relied on natural language processing techniques to recognize similar words and phrases and compared each model in our database to the bills that lawmakers had introduced. These comparisons were powered by the equivalent of more than 150 computers, called virtual machines, that ran nonstop for months. Even with that computing power, we couldn't compare every model in its entirety against every bill. To cut computing time, we used keywords - guns, abortion, etc. The system only compared a model with a bill if they had at least one keyword in common. The team then developed a matching process that led to the development of an updatable, public-facing tool that reporters and members of the public can use to identify not only past bills but future model bills as they are introduced, while the bills are still newsworthy.
作品最难完成的部分: It’s hard to overstate how resource-intensive this analysis was. This was our first foray into natural language processing. We had to compare one million bills — each several pages long, with some up to 100 pages in length — to each other. Computationally, scale bought with a lot of complexities. We had to go deep into understanding how to deploy some of the software we used at scale and solve the problems we faced along the way. We spent tens of thousands of dollars on cloud services. We had to re-run this analysis every time we made changes to our methodology — which we did often. The resulting analysis and reporting took more than six months to put together. We obtained metadata on more than 1 million pieces of legislation from all 50 states for the years 2010 through 2018 from a third-party vendor, Legiscan. We also scraped bill text associated with these bills from the websites of state legislatures.In addition, we pieced together a database of 2,000 pieces of model legislation by getting data from sources, downloading data from advocacy organizations and searching for models ourselves. This was done either by identifying known models and trying to find the source or finding organizations that have pushed model bills and searching for each of the models for which they have advocated.
作品值得学习的地方: The power of collaboration. CPI and USA TODAY/Arizona Republic built two analysis tools to identify model language, using two different approaches. USA TODAY's efforts found at least 10,000 bills almost entirely copied from model language that were introduced in legislatures nationwide over the last eight years. CPI’s tool worked to identify common language in approximately 60,000 bills nationwide to flag previously unknown model legislation. Together the tools allowed for analysis of success from identified model bills and enabled identification of new model legislation. The computer comparisons, along with on-the-ground reporting in more than a dozen states, revealed that copycat legislation amounts to the nation’s largest, unreported special-interest campaign. Model bills drive the agenda in states across the U.S. and influence almost every area of public policy.
作品链接:
- https://www.usatoday.com/in-depth/news/investigations/2019/04/03/abortion-gun-laws-stand-your-ground-model-bills-conservatives-liberal-corporate-influence-lobbyists/3162173002/
- https://publicintegrity.org/politics/state-politics/copy-paste-legislate/the-multistate-push-to-let-dealers-get-away-with-selling-you-a-defective-car
- https://www.usatoday.com/in-depth/news/local/arizona/2019/06/19/abortion-laws-2019-how-heartbeat-bills-passed-ohio-missouri-more/1270870001/
- https://web.model-legislation.apps.fivetwentyseven.com/
- https://www.usatoday.com/in-depth/news/investigations/2019/07/17/islam-sharia-law-how-far-right-group-gets-model-bills-passed/1636199001/
- https://www.usatoday.com/in-depth/news/investigations/2019/10/02/catholic-church-boy-scouts-fight-child-sex-abuse-statutes/2345778001/
- https://publicintegrity.org/politics/state-politics/copy-paste-legislate/big-tobaccos-surprising-new-campaign-to-raise-the-smoking-age
最佳数据驱动报道(小型新闻机构)
得奖作品: Made in France
机构: DISCLOSE
国家: 法国
作者: Mathias Destal, Michel Despratz, Lorenzo Tugnoli, Livolsi Geoffrey, Aliaume Leroy
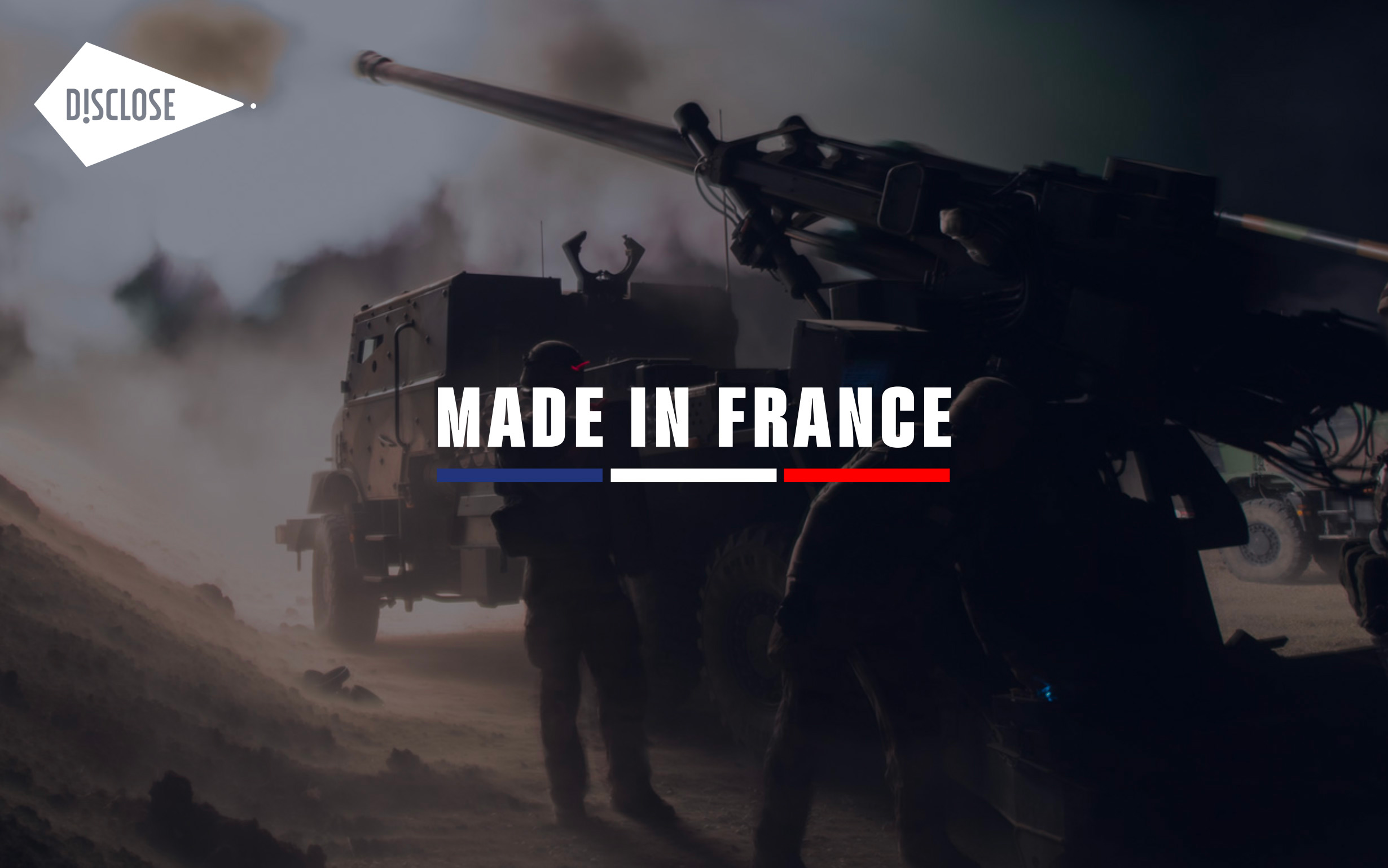
评审团评语: “Made in France” is an investigation that proves beyond doubt that powerful journalism is born at the intersection between traditional reporting, advanced data analysis and courage. The Disclose team used highly confidential documents as a base layer to build up an exposé that brought to light the extent of France’s military involvement in the Yemen conflict. This is hard and risky work in the public interest, and it was greatly augmented by the advanced data journalism techniques that the team employed to mine, map, fact-check and display its findings.
机构类别: 小型机构
发表日期: 15 Apr 2019
作品简介: Following six months of investigation, Disclose reports on how french made weapons sold to Saudi arabia have been used against the civilian population in the Yemen war. Disclose used an unprecedented leak of secret documents and used OSINT research and data analysis to establish French responsibility for the war in Yemen. An investigation combining both human sources, secret documents and open source information, using satellite imagery to track French weapons in Yemen and their impact.
作品效果与影响力: The investigative story was published simultaneously on five media in France. The project has placed the question of France's arms sales to Saudi Arabia at the center of the political and civil debate. The Minister for the Army and the French Minister for Foreign Affairs were heard by the parliament. The information has demonstrated the lies of the French government on the ongoing arms exports to Saudi Arabia. Dozens of NGOs have called on the government to stop arms deliveries to Saudi Arabia and several public demonstration take place in France against arms deliveries. A month after the revelations, the government, under pressure from public opinion, had to cancel two arms deliveries to Saudi Arabia, for the first time since the Algerian war. In January 2020, the government suspended the delivery of bombs to Saudi Arabia.
技术/科技: We used satellite images to prove the presence of French weapons used in the Yemen War. We watched dozens of videos found on official social accounts, which we then geolocated using satellite views. So we were able to prove the presence of French military equipment in Yemen. We use open data from the Yemen Data Project to know in order to know the number of civilian victims in the firing range of French hotwizer and by calculating their range from public information given by the manufacturing companies. With this information, we were able to find possible evidence of civilian deaths related to these weapons. We used satellite images, webcam and data from Marine Traffic to retrace the course of a boat carrying arms from France to Saudi Arabia. We have also analysed the details of 19,278 aerial bombing raids recorded between March 26th 2015 and February 28th 2019.The results: these show that 30% of the bombing raids were against civilian targets. The intent of the coalition was clearly to destroy infrastructures that are essential for the survival of Yemen’s population of 28 million people. We geolocated all this bombing on map and find evidence on social network of the bombing.
作品最难完成的部分: The "Made in France" project had for finality to investigate a sensitive topic covered by military secret in France and whose investigation on the ground was made difficult or even impossible due to the ongoing conflict. The objective was despite these problems to conduct an investigation into the sale of weapons and their use in the war in Yemen with public data and open source information. The hardest part of this project was to verify and publish this secret documents. We want not only to publish a secret document but use the same intelligence tools used by the French military to prove the implication of our weapons in the war in Yemen. The hardest part was to disclose the route of arms deliveries by boat, the information of which is nevertheless classified as military secret. We wanted to show that only with open source information we could investigate hidden matters. "Made in France" project is an unprecedented multi-long format that brings data journalism to one of the most difficult areas of investigative journalism.
作品值得学习的地方: This project is a demonstration that we can investigate on arms deliveries only with public data, that we can investigate war grounds from a computer screen. But data journalism is not a dehumanized journalism, because journalism needs sources and whistleblower to have information. Data journalism can be a powerful means of investigation also on the more sensitive topics like war and arms trade.
作品链接:
- https://made-in-france.disclose.ngo/en/
- https://youtu.be/BKUi1HmaJL0
- https://theintercept.com/2019/05/17/france-takes-unprecedented-action-against-reporters-who-published-secret-government-document/
最佳新闻应用
得奖作品: HOT DISINFO FROM RUSSIA (Topic radar)
机构: TEXTY.org.ua
国家: 乌克兰
作者: Nadiia Romanenko, Nadja Kelm, Anatoliy Bondarenko, Yuliia Dukach
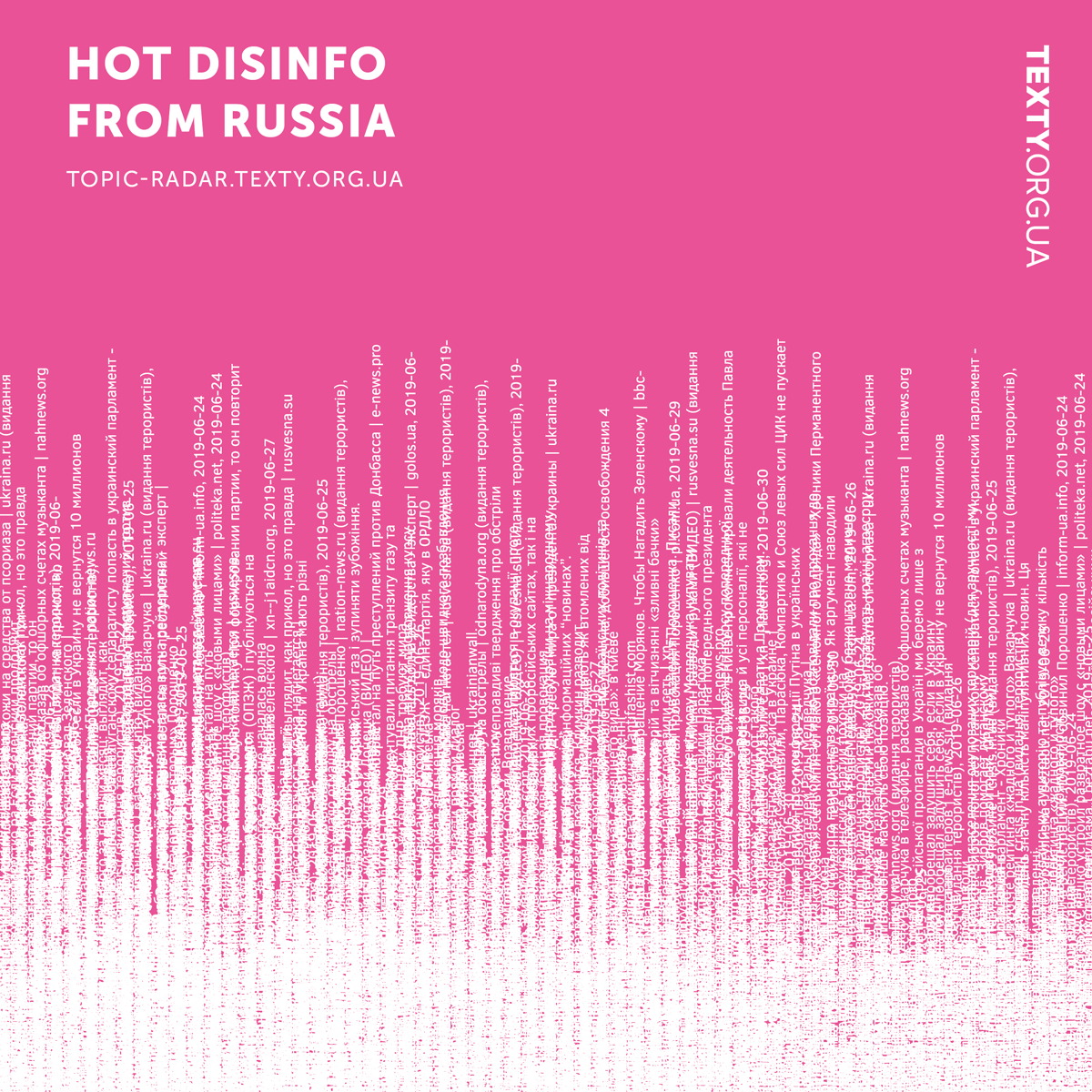
评审团评语: Disinformation can play an important role in international politics, and more so when there is limited public awareness about the interference. The jury is delighted to find an app developed to address that in Ukraine. The tool tracks the content and intensity of Russian disinformation narratives and manipulative information in online media, and shows an overall dynamic as a result. As the first of its kind for Russian and Ukrainian languages, it allows user engagement in different ways, visually as an interactive dashboard, analytically through weekly posts, and functionally by offering a browser add-on to help individual citizens identify manipulative content. The project shows exactly what a great news app should do, which is to empower users to find their own narrative and make their own judgement within a larger dataset, and it is addressing some of the most critical challenges for journalism today.
机构类别: 小型机构
发表日期: 7 Aug 2019
作品简介: TEXTY developed the data acquisition&analysis platform and dashboard tool https://topic-radar.texty.org.ua which shows an overall dynamics of topics of Russian disinformation in manipulative news. We are doing an NLP on thousands of news per week to detect manipulative ones, group them by topics and meta-topics to show on interactive dashboard. We also publish weekly reviews (21 so far), based on the results of analysis. In addition we developed "Fakecrunch" add-on based on the same platform (for Chrome and Firefox). It automatically signals to users about manipulative content and could be used to collect suggestions about possible low quality/fake/manipulative news items.
作品效果与影响力: The project is aimed to track the content and intensity of Russian disinformation narratives and manipulative information in online media. It raises awareness of government bodies, civil society organizations, journalists and experts on major disinformation themes that are being pushed by Russia at any given week. Just one example: Dmytro Kuleba, Deputy prime minister of Ukraine, mentioned this project as an illustration of the huge level of Russian disinformation flowing to Ukraine. This quantitative approach allows us to overview and to zoom-in, from top to bottom, of the vast propaganda landscape and to track topics in different periods of time. Starting from May 2019, 21 weekly reviews, based on the project, were published. Each review illustrated key narratives of manipulations, which our application determined. Average audience engagement for each publication on texty.org.ua was about 8,000 users. Other media used to share our reviews, as well as some bloggers and influencers. Also we got positive feedback and mentions of this news application from international experts, for example Andreas Umland (German), Lenka Vichova (Czech republic). In words of Maciej Piotrowski, from Instytut Wolności in Warsaw, Poland: "Useful information. Sometimes we share it in our materials in Instytut Wolności, sometimes used for analysis. Longtime tracking is useful to see the full picture." After many requests about additional features we decided to develop version 2 of the application. It will be published in April 2020 (approximate date) and we’ve freezed data updates until the new version arrives.
技术/科技: Data was downloaded from sites' RSS feeds or links on their Facebook pages. Preprocessed data about news items stored in PostgreSQL. Each text was prepared for analysis: tokenized (divided into language units — words and punctuation marks), lemmatized for topic modeling. Custom Python scripts were used to obtain (Scrapy), process and store data. Each news item was then evaluated by an improved version of our manipulative news classifier ( ULMFiT based model for Russian and Ukrainian languages, created by TEXTY back in 2018, programmed in Pytorch/fast.ai). This model is available from our github. It estimates the likelihood that the news contains emotional manipulation and/or false argumentation. Selected manipulative news, ~3,000 pieces per week on average, was broken down into topics by automatic topic modeling (NMF algorithm). We edited the resulting news clusters manually: combined similar topics, discarded irrelevant or overly general clusters. Each subtopic in our news application is also illustrated by a sample of titles from news which belong to it to let new readers know what it is about.
作品最难完成的部分: For our best knowledge, this is the first such tool & whole pipeline for Russian and Ukrainian languages. The main challenge was to retrieve accurate topics and track them over time. Topic modelling was made using NMF, an unsupervised method of clusterization. Results are less accurate compared to supervised learning, when the model is trained using humal labels. But we cannot train topic classifier since we do not know all the topics in news and cannot easily update supervised model if the news agenda changes. So we have to keep using unsupervised NMF solution. Topics for the week are reviewed by analysts, as well as improved by rules to fix possible errors of unsupervised topic modelling. A lot of manual work is the hard part of this project. Because we detect topics in weekly samples of news, we have to aggregate them for dashboard to track topics for longer periods. We addressed this challenge by hierarchical NMF, namely clusterized weekly clusters. Meta-topics in the dashboard were first clusterized and reviewed by analysts so that each weekly topic relates to one meta-topic on the dashboard. Aggregation of clusters from different models is not well-studied and a great part of it is done manually.
作品值得学习的地方: Long-term tracking of disinformation makes it possible to see what topics are most important for the Russian authorities, who is the biggest irritant to them, and what they plan to do in the future in Ukraine. One of the conclusions of our analysts is evidence that there are entire array of manipulative news from Russia which can be logically combined under the umbrella name of “Failed state” (related to Ukraine). The purpose of this campaign is obvious: it aims to create an image of Ukraine as a non-state, an artificial state entity that arose against historical logic. We are considering the dashboard as a usable tool for further research by analysts, and Fakecrunch add-on as a usable tool for online-readers in their everyday "life". Other journalists got the source for their materials. General public got evidence-based tool for media literacy and for self-control in social media. Lenka Vichova, Czech Republic: "Many of these messages enter not only the information field of Ukraine, but also to Czech and Slovak media sphere. So it is core to know and be prepared. I use your reviews, when working on my own analytical articles and also in comments for Czech and Slovak media."
作品链接:
- https://topic-radar.texty.org.ua
- http://texty.org.ua/mod/archive/tag.php?tag=disinfomonitor
- https://fgz.texty.org/
最佳数据可视化报道(大型新闻机构)
得奖作品: See How the World's Most Polluted Air Compares With Your City's
机构: The New York Times
国家: 美国
作者: Nadja Popovich, Blacki Migliozzi, Karthik Patanjali, Anjali Singhvi and Jon Huang
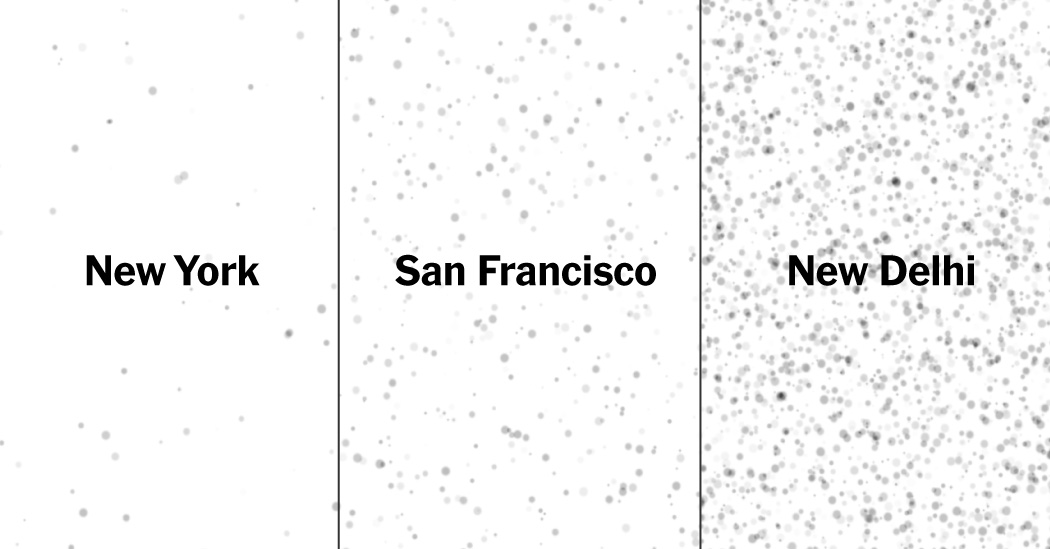
评审团评语: This data visualization is effective and pushes the limit in explaining a complex and important topic, making it easy to understand in a detailed and granular way the public health hazard of air pollution that causes millions of deaths and illnesses worldwide. It combines the best of beauty, storytelling and interactive features. Users can learn via preset examples, or extract and produce their own stories and comparisons. In mobile, it excels, including AR experimentation that brings data to life. The visualization builds empathy through data, using case studies of polluted air that have recently made news and making visible the invisible. The combination of precision in data usage with the best of visual digital technologies and users' interaction, works perfectly to tell this complex story in an engaging and meaningful way.
机构类别: 大型机构
发表日期: 2 Dec 2019
作品简介: Outdoor particulate pollution known as PM2.5 is responsible for millions of deaths around the world each year and many more illnesses. We created a special project that visualizes this damaging but often invisible pollution. The interactive article allows readers to (safely) experience what it’s like to breathe some of the worst air in the world in comparison to the air in their own city or town, giving them a more personal understanding of the scale of this public health hazard.
作品效果与影响力: This air pollution visualization project was one of The Times' most-viewed stories of the year, garnering well over a million page views in a single day. It also had some of the highest reader engagement. Readers took to social media, unprompted, to share the air pollution averages for their own city as well as screenshots of the project’s visualizations, and to express concern over recent upticks in air pollution. Making air pollution more tangible to the general public is especially important today, as air quality in the United States has worsened after decades of gains, while much of the world’s population continues to breathe high levels of pollution. At the same time, it is becoming more clear that air pollution affects human health at ever more granular scales. Experts from the public health community, including the United Nations and WHO, have reached out about using the project for educational purposes.
技术/科技: Particle visualization and charts: The data analysis was done using Python. Visuals in the story were created using WebGL and D3. Augmented reality version: The AR experience was created using Xcode and Apple SceneKit. (The AR scene being responsive to data was created using Swift in Xcode.) Please not that the AR version is only available on the New York Times app and on iPhones due to technological constraints of the Android operating system. Map: The map was rendered by converting netCDF files using R and gdal. The animation was done using Adobe's After Effects and Illustrator.
作品最难完成的部分: We wanted the project to build empathy through data by connecting people's own experience (what average air pollution is like in their own city) to various case studies of polluted air that have recently made news. To achieve that, we strove to make sure the visualization had the right feeling of movement in space to evoke polluted air, while still reflecting that it a data visualization rather than an accurate reflection of what pollution might look like at a specific place and time. We went through many ideas for how to represent this pollution – as particles, as haze, etc. – and many ways to show it to our audience. The end goal: Walking the line between what is scientifically accurate while also allowing people to feel a natural connection between the viz and the subject being visualized (pollution).
To ensure scientific accuracy, we ran our visualization ideas past half a dozen experts who study particulate matter pollution in order to best decide how to show these damaging particles. In the end we settled on a deceptively simple presentation: Filling up your single screen (or room in AR) with particles as you scroll (or tap) in order to create a sense of "filling" your lungs with this sort of air. Our readers' reactions to the piece suggest that we got the balance right.
作品值得学习的地方: One lesson we hope people will take away is that it is possible to create emotional connections to data through visualization. We built the story introduction so that readers become the central character, allowing them to use their own experience of polluted air as a benchmark by which to judge and understand the scale of pollution elsewhere. That builds a deeper understanding of the issue at stake than just showing data for far-away places they may have never visited. On the more technical side, many people commented on the project's innovative use of augmented reality. The Project leveraged AR to make something that is all around us but often invisible actually visible in 3D space. Previously, experiments with AR at the Times and in other newsroom mostly consisted of placing objects into space (such as the Times' Lunar landing project) or creating a novel 3D space for exploration (such as the Guardian's augmented reality project that allowed users to experience what being in solitary confinement is like).
Selected praise for the AR experience:
- "This is easily the most compelling use of augmented reality I've ever seen in a news context." – Chris Ingraham, Washington Post
- "I've been always (and I still am largely) skeptical about the application of #AR and #VR especially in #dataviz but this made me change my mind: it's all about the way it relates to our perception and experience of the world around us." – Paolo Ciuccarelli, prof at NortheasternCAMD
作品链接:
最佳数据可视化报道(大型新闻机构)
荣誉奖:Why your smartphone is causing you ‘text neck' syndrome
机构: 南华早报
国家/地区: 中国香港
作者: Pablo Robles
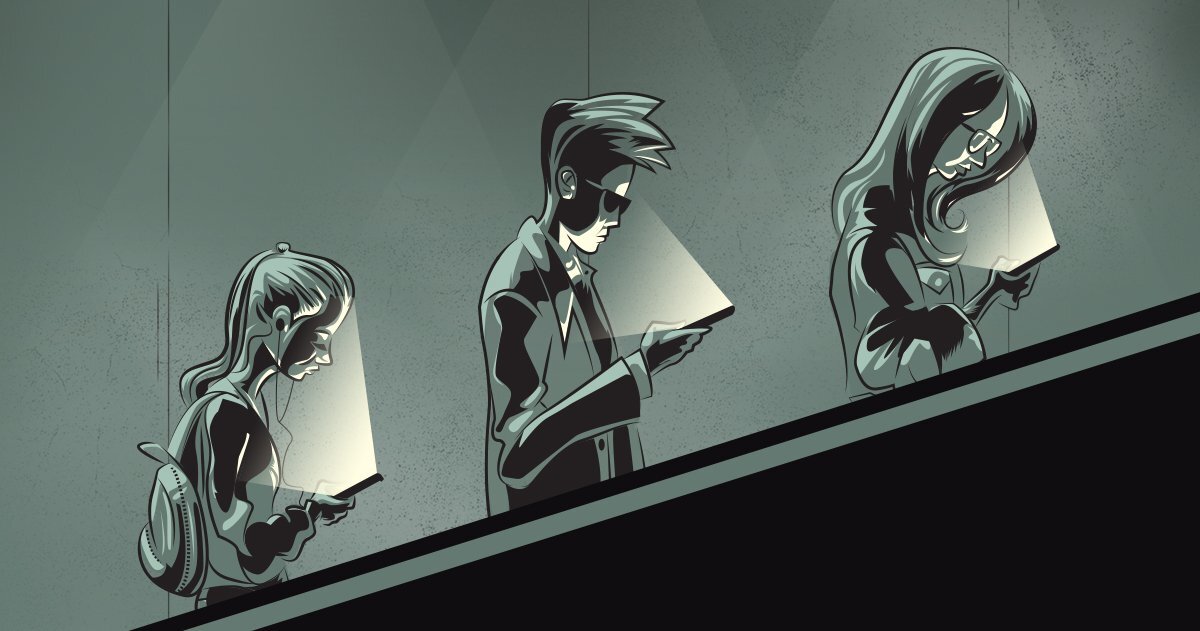
评审团评语: The jury decided to recognize "Why your smartphone is causing you ‘text neck’ syndrome" with an honourable mention, based on its technical excellence and engaging use of graphics. The project's narrative was clear and easy to follow and the interactive and non-interactive images interspersed among the text meant there was always something interesting to engage the reader. There were also a wide range of visual techniques used from static graphics to interactive ones, to annotated video. While there was some debate about the data behind the "text neck" syndrome the panel recognised the excellent presentation of the narrative as a whole.
机构类别: 大型机构
发表日期: 25 Jan 2019
作品简介: Mobile phones are now generally seen as essential to our daily lives. Texting has become the way most of us communicate and has led to rapidly increasing numbers of people suffering from 'text neck'. For our visualisation, “Why your smartphone is causing you ‘text neck’ syndrome” we researched how the angle of your neck when you look at your phone can effectively increase the weight of your head by up to 27kg. This in turn can damage posture and, if you text while walking, expose you to all kinds of dangers.
作品效果与影响力: This data visualisation caused much debate on social media and was translated into Spanish and republished by artesmedia.com
技术/科技: We collected data about mobile phone internet access by country. Using dataviz and diagrams, graphics and our own video footage we detailed how extensive mobile phone use leads to curvature of the spine. We also recorded more than 10 hours of video to analyse how people use their mobile phones in Hong Kong when walking and crossing streets. The data confirmed the study made by the University Of Queensland. We also use data research to explore mobile phone addiction and to explain how users ‘zone out’ on their phones. We hope that our innovative storytelling will make readers aware of their own habits and understand how their actions impact those around them as well as themselves.
作品最难完成的部分: We also recorded more than 10 hours of video footage of mobile phone use on the streets of Hong Kong to corroborate an academic study from the University Of Queensland. We pepper the story with short videos to demonstrate how peripheral vision is restricted when using mobile phones, how your gait changes and to illustrate the dangers people pose while texting and walking in the street and using public transport.
作品值得学习的地方: We believe this data visualisation helps make readers aware of their own habits and understand how their actions impact those around them as well as themselves.
作品链接:
最佳数据可视化报道(小型新闻机构)
得奖作品: Danish scam
机构: Pointer (KRO-NCRV)
国家: 荷兰
作者: Peter Keizer, Wendy van der Waal, Marije Rooze, Jerry Vermanen, Wies van der Heyden
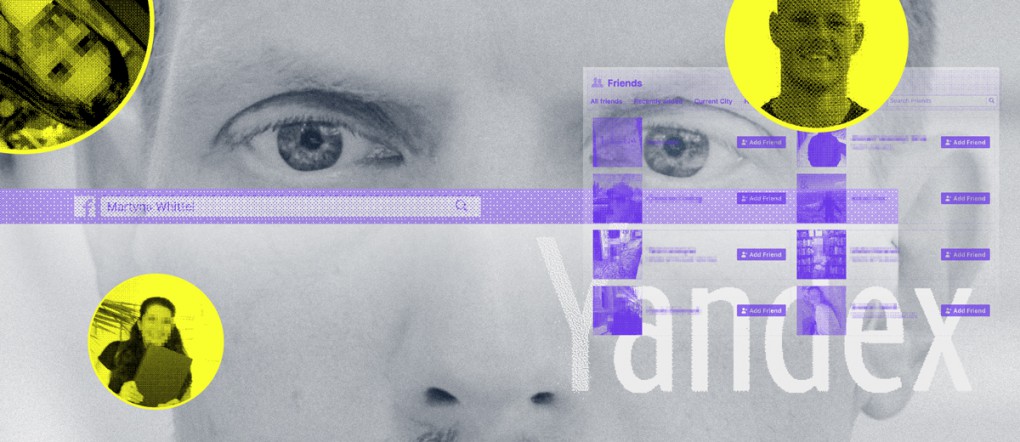
评审团评语: Dutch journalist and data researcher, Peter Keizer places readers in the driver’s seat on a journey into the murky world of identity theft. The colourful and bold layout is clean and simple and houses a detective story that analyzes emails and websites, screens companies and traces the Danish scammers’ employees via social media to the Philippines. Keizer uncovers 134 cases of identity theft and contacts some of the victims. “It’s my photo and name, but I didn’t know anything about it. I don’t like that at all. But I wonder how I can deal with those boys now,” complains one stooge. The whodunit format resonates with the public by showing how vulnerable all of us are to being scammed unwittingly. This piece might not be what we traditionally think of as data visualization but instead broadens the remit by transforming information into a visual context to tell a compelling story.
机构类别: 小型机构
发表日期: 12 Jul 2019
作品简介: One day in 2019, we received an obvious spam email in which we were asked to publish a guest blog on our website. Normally we would delete this, but after a follow-up email we became curious on how this scam works. We decided to find out for ourselves. With the information in the email, we searched and found an elaborate network of two Danish scammers and at least 134 persons whose identities were stolen. We made an article in which we put you in the driver seat of our lead investigator.
作品效果与影响力:After our first publication and visualisation, we made a TV broadcast 4 months after the fact. We translated our online production to TV, instead of making an online production from our programme. In the TV broadcast, we also filmed our investigator’s screen and tried to do everything from behind our laptop. During this second investigation, we discovered that the Danish guys improved their scam. They AI generated faces to fake reviews, contact persons and sell their content. So we made a second visualisation in which we explain how you can recognize this more sophisticated scam. We tried to contact as many victims as possible. Most of them didn’t know their identities were used for this scam.
技术/科技:We didn’t want to tell this story in a familiar way: the most exciting part is discovering the answers step by step. So we searched for a way to translate a research on desktop to your mobile screen. We used OSINT techniques like reversed image search, Wayback Machine searching, Google Dorks, searching in chambers of commerce, digital forensics to find outgoing url’s, etc. to reveal the intricate and complicated network behind this scam. We also made our own database of persons whose identities were stolen. We needed to know how many people were involved, and if they knew anything about this scam. The most difficult person to find was Martyna Whittell, the fake identity of our emailer. She used photos of an existing person. We found the real ‘Martyna’ (her name is Mia) by geolocating her photos: we found a photo on a campus in Aalborg through a Starbuck coffee cup and a concert photo through the background of a Take That reunion tour. We eventually used face recognition in Yandex to find her friend on a group photo, and searching her friend list for a photo that looked like Mia.
作品最难完成的部分: The hardest part of our research was finding Mia. We could find a lot of breadcrumbs online to reveal the scam(mers), but finding our main victim was difficult. Also, making a visualisation that works on mobile and puts you in the seat of our investigator was a real challenge. We could make a direct analogue with a desktop computer, because of the orientation of your screen. Forcing users to rotate their screens would be a step in which most people would back-out and quit. We found a way in which we made our own screens with illustrations. This also works great in this example, because we needed to anonymize almost everyone. We translated the story to English because this story is not only interesting for Dutch readers.
作品值得学习的地方: The most important lesson is never to take anything for granted: a good investigative story can hide itself in an ordinary spam email you get every day. Also, making your own databases and being well-versed in digital research techniques is an essential part of modern investigative journalism. The translation from desktop to mobile was a successful, in our opinion. We found that a lot of readers scrolled to the end of our story.
作品链接:
- https://pointer.kro-ncrv.nl/artikelen/het-verhaal-achter-een-identiteitsroof#lang=en
- https://pointer.kro-ncrv.nl/artikelen/dozens-of-identities-stolen-by-danish-online-entrepreneurs
- https://pointer.kro-ncrv.nl/artikelen/our-tricks-for-identifying-victims-of-identity-fraud
- https://pointer.kro-ncrv.nl/artikelen/online-oplichters-gaan-vaak-vrijuit
- https://pointer.kro-ncrv.nl/artikelen/terugkijken-hoe-een-mysterieus-mailtje-ons-leidt-naar-online-oplichters-in-de-filipijnen
- https://pointer.kro-ncrv.nl/artikelen/hoe-ontmasker-je-een-online-oplichter-in-7-stappen#lang=en
最佳创新奖 (大型新闻机构)
联合得奖作品:AP DataKit: an adaptable data project organization toolkit
机构: The Associated Press
国家: 美国
作者: Serdar Tumgoren, Troy Thibodeaux, Justin Myers, Larry Fenn, Nicky Forster, Angel Kastanis, Michelle Minkoff, Seth Rasmussen, Andrew Milligan, Meghan Hoyer, Dan Kempton
评审团评语: AP’s DataKit is an innovation will change the way many data reporters/editors/teams work and will undoubtedly have a profound impact on the data journalism community at large. Not only is it a tool that can help data journalists work more efficiently more collaboratively, it is a platform that is already being extended by contributors outside of AP. If data journalism is the imposition of structure and reproducibility with a journalistic bent, DataKit promises to be the tool that enforces that structure and enables more efficiency and collaboration for data teams in every newsroom.
机构类别: 大型机构
发表日期: 12 Sep 2019
作品简介: AP DataKit is an open-source command-line tool designed to help data journalists work more efficiently and data teams collaborate more effectively. By streamlining repetitive tasks and standardizing project structure and conventions, DataKit makes it easier to share work among members of a team and to keep past projects organized and easily accessible for future reference. Datakit is adaptable and extensible: a core framework supports an ecosystem of plugins to help with every phase of the data project lifecycle. Users can submit plugins to customize DataKit for their own workflows.
作品效果与影响力: The AP open-sourced its project-management tool, DataKit, in September of 2019. Our data team has used it internally for two years now on every single analysis project we've done. Its purpose is simple, yet sophisticated: With a few command-line directions, it creates a sane, organized project folder structure for R or Python projects, including specific places for data, outputs, reports and documentation. It then syncs to GitHub or Gitlab, creating a project there and allowing immediate push/pull capabilities. Finally, it syncs to S3, where we keep our flat data files and output files; and to data.world, where we share data with AP members. DataKit's release came at ONA and attracted the attention of roughly 60 or so conference attendees, many of whom returned to their classrooms and newsrooms to try it out. It has been adopted by individual users, by the data analysis team at American Public Media, and is in use in some data journalism classes at University of Maryland and University of Missouri. We'll have another install party for interested data journalists at NICAR in March. Interestingly, the project has also had several open-source contributions from the journalism community. Several journalists have built additional plug-ins for DataKit -- for instance, one coder wrote a plugin to sync data to Google Drive. The impact of DataKit is fundamental: it allows us to move quicker and collaborate better, by creating immediate and standardized project folders and hook-ins that mean that no data journalist is working outside of replicable workflows. Data and code gets synced to places where any team member can find them; and each project looks and acts the same. It creates a data library of projects that are well-documented, all in one place and easy to access.
技术/科技: DataKit is an extensible command-line tool that's designed to automate data project workflows. It relies on core Python technologies and third-party libraries to allow flexible yet opinionated workflows, suitable for any individual or team. The technologies at the heart of DataKit are: [Cliff](http://docs.openstack.org/developer/cliff/) - a command-line framework that uses Python's native setuptools entry points strategy to easily load plugins as Python packages. * [Cookiecutter](https://github.com/cookiecutter/cookiecutter) - a Python framework for generating project skeletons Through the cookiecutter templates, DataKit creates a series of folder and file structures for a Jupyter notebook or an RStudio project. It also configures each project to sync to the proper gitlab and S3 locations, and loads specific libraries, dependencies and templated output forms (such as an RMarkdown customized to match AP design style). The AP has built four plug-ins: for Gitlab and GitHub; for S3 and for data.world. Other open-source users have since built additional plug-ins to customize DataKit to their workflows, such as syncing to additional data sources (Google Drive) and outputs such as Datasette.
作品最难完成的部分: The most difficult part of the project was creating clear, concise documentation that would help others use our open-source software. We had never open-sourced something so ambitious before, and were put in the position of anticipating others' uses (we created a GitHub plug-in despite our team not using GitHub regularly) and others' pain points in understanding, installing and using DataKit. We created DataKit to scratch our own itch -- to make our team work better, faster and with more precision and control. Having DataKit means we spend less time every day handling the messy, boring parts of a project -- finding old files, creating working directories -- and more time on the serious data analysis work we need to be doing. The AP is a collaborative news cooperative, and in that spirit, it made sense this year to fully open-source one of our team's most powerful tools to share it with others. One of our goals is to make data more accessible to other newsrooms, and DataKit we hope does this by taking away some of the barriers to getting to an analysis and sharing data.
作品值得学习的地方: Creating standardized workflows across a data team leads to quicker, more collaborative and stronger work. Data workflows can be notoriously messy and hard to replicate -- Where are the raw data files stored? What order do you run scripts in? Where's the documentation around this work? Is the most recent version pushed up to GitHub? Can anyone beside the lead analyst even access data and scripts? -- and DataKit was built to fix that. The thing AP's Data Team would like others to come away with is that we don't all have to use these messy, irreproducible and bespoke workflows for each project that comes across our desk. Creating a standardized project structure and workflows creates sanity -- through DataKit we at the AP now have an ever-growing library of data and projects that we can grab code from, fork or update when needed -- even on deadline. We can also dip into each other's projects seamlessly and without trouble: One person's project looks like another's, and files and directories are in the same places with standardized naming conventions and proper documentation. DataKit simply lets analysis teams work better, and faster, together. One real-life example from 2019: When we received nearly a half billion rows of opioid distribution data this summer, and were working on deadline to produce an analysis and prepare clean data files to share with members, we had six people working concurrently in the same code repository with no friction and no mess. The AP landed an exclusive story -- and shared data files quickly with hundreds of members -- thanks to DataKit.
作品链接:
- http://datakit.ap.org/
- https://www.rjionline.org/stories/ap-datakit-intro
- https://ona19.journalists.org/sessions/23627451/#audio
- https://www.poynter.org/tech-tools/2019/data-journalism-solves-big-problems-but-its-an-organizational-mess-a-new-tool-from-the-ap-aims-to-fix-that/
- https://github.com/associatedpress/datakit-core
最佳创新奖 (大型新闻机构)
联合得奖作品:Zones of Silence
机构: El Universal
国家: 墨西哥
作者: Esteban Román, Gilberto Leon, Elsa Hernandez, Miguel Garnica, Edson Arroyo, César Saavedra, Jenny Lee, Dale Markowitz, Alberto Cairo
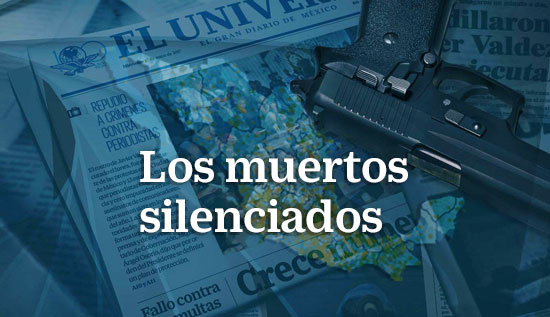
评审团评语: How do you measure the something that isn't happening? What if the main cause of concern isn't noise but silence? El Universal asked that question about the falling levels of coverage of homicides in Mexico, working on the hypothesis that journalists have been intimidated and harassed into silence. By comparing murder statistics with news stories over time, they were able to show where, and by how much, the troubling silence was growing.
机构类别: 大型机构
发表日期: 13 Jun 2019
作品简介: Violent organized crime is one of the biggest crises facing Mexico. Journalists avoid becoming a target, so they choose to stay quiet to save their lives. We set out to measure this silence and its impact on journalism. To do so, we used artificial intelligence to quantify and visualize news coverage and analyze the gaps in coverage across the country. To measure the degree of silence in each region of the country, we created a formula that allows us to see the evolution of this phenomenon over time.
作品效果与影响力: Something akin to a code of silence has emerged across the country. We suspected that there were entire regions where journalists were not reporting on the violence, threats, intimidation and murder that were well known to be part of daily life. This was confirmed by journalists who sought for us after the story was released, to tell us they have been facing this problems. In collaboration with them, now we are preparing a second part of this story, to focus on the patterns that lead to agressions. Hopefully this will lead us to some kind of alert when certain conditions (of news coverage and crimen) are present in regions of our country.
技术/科技: Our first step was to establish a process to determine the absence of news. We explored articles on violence to understand how they compare to the government's official registry of homicides. In theory, each murder that occurs ought to correspond with at least one local report about the event. If we saw a divergence, or if the government's reports were suddenly very different from local news coverage, we could deduce that journalists were being silenced. Early on, sorting through news articles seemed impossible. We knew we needed to find a news archive with the largest number of publications in Mexico possible so we could track daily coverage across the country. Google News' vast collection of local and national news stories across Mexico was a good fit. The effort required us to identify the difference between the number of homicides officially recorded and the news stories of those killings on Google News. This required machine learning algorithms that were able to identify the first reported story and then pinpoint where the event took place. With that information, we were able to connect reported events by media with the government's reports on homicides across more than 2400 municipalities in Mexico. Finally, to measure the degree of silence in each region of the country, we created a formula that allows us to see the evolution of this phenomenon over time. The resulting data shows a fascinating mix of falls or peaks in unreported deaths, which coincide with events such as the arrival of new governments or the deaths of drug dealers. Further investigation will allow us to explain these connections.
作品最难完成的部分: The hardest part was creating the "formula for silence" to measure the degree of non reported homicides along the country. There are many variables behind the reason why there aren't as much articles as homicides in each region. So, in order to be sure the discrepancy was linked to violence and killings we had to rule out or include segments of data along the way. This was extremely hard to do with machine learning, because words in spanish that are usually used to represent this kind of coverage, are also synonyms for other things. We had to validate (manually) a lot of the initial reports until we had a well validated sample of results. This took us half a year. Then we felt lost due to the amount of variables we had in our hands (disparity between events reported and published stories; matching stories reporting one single event by different websites; the uncertainty of internet penetration in all parts of the country and its evolution over time within the 14 years we analyzed...). Luckly, the interdisciplinary nature of our team (with economists, programmers, data experts, designers and journalists) helped us to find an answer that we felt was truly accurate.
作品值得学习的地方: No matter how hard it is to measure a problem, there is always a way to do it, even if its not what you thought you would find in the beginning.
作品链接:
- https://zonas-de-silencio.eluniversal.com.mx/
- https://www.eluniversal.com.mx/nacion/sociedad/en-10-estados-guardan-silencio-sobre-homicidios
- https://www.eluniversal.com.mx/nacion/sociedad/la-prensa-que-ya-no-habla-de-muertos
- https://zonas-de-silencio.eluniversal.com.mx/metodologia.html
最佳创新奖 (小型新闻机构)
得奖作品: Funes: an algorithm to fight corruption
机构: OjoPúblico
国家: 秘鲁
作者: Gianfranco Rossi, Nelly Luna Amancio, Gianfranco Huamán, Ernesto Cabral, Óscar Castilla
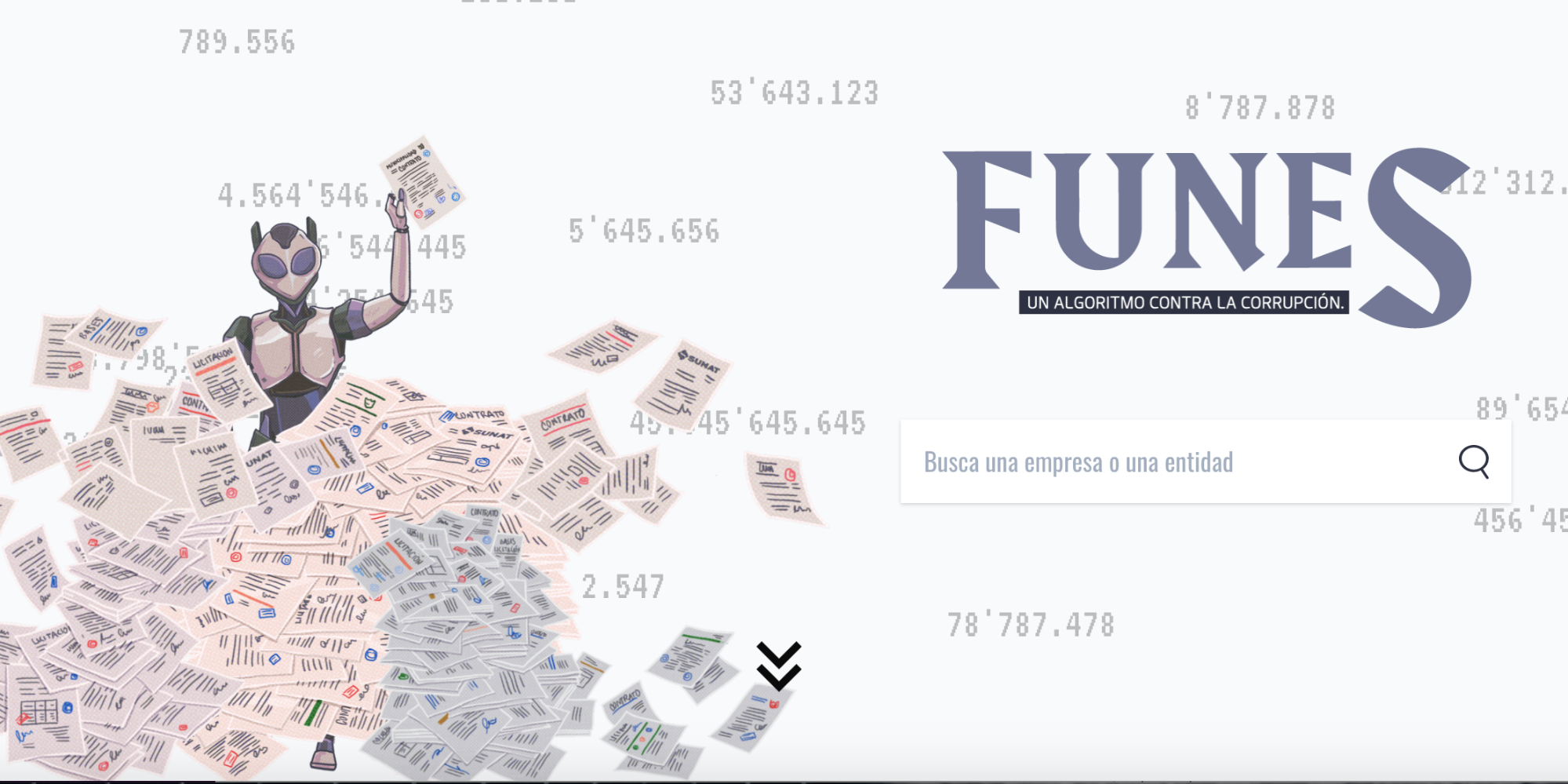
评审团评语: As more and more potentially newsworthy documents become routinely available online as digital data, classifying this deluge and prioritising reporters’ attention is becoming one of data journalism’s major challenges. The “Funes” tool from Peru’s OjoPúblico shows that even relatively small organisations can develop algorithms to help tackle this problem for specific types of documents. Funes adapts a model a contracting risk model developed in Europe to the Peruvian context. Using data scraped from five public databases, the algorithm analysed hundreds of thousands of Peruvian public procurement documents. Using a linear model, it combines 20 risk indicators — such as recently founded contractors or uncontested bids — to flag potentially corrupt contracts. It resulted in a large volume of cases for OjoPúblico and regional media partners to investigate as well as an interactive interface for readers, providing an excellent pioneering example of the sort of automated story discovery tools several judges said they expect to become an increasingly important area of investigative computational journalism.
机构类别: 小型机构
发表日期: 25 Nov 2019
作品简介: Funes is an algorithm that identifies corruption risk situations in public contractings in Peru. The research project began to take shape in February 2018 and its development began in September of the same year. For 15 months a multidisciplinary team - integrated by programmers, statisticians and journalists - discussed, analyzed, built databases, verified the information and developed modeled an algorithm we call Funes, as the memorable protagonist of the Argentine writer Jorge Luis Borges. The algorithm rates a risk score for each contract process, entity and company. With that information journalists can prioritize their investigations.
作品效果与影响力: The project was developed in the context of the fiscal investigations of the Lavajato case, which involves the payment of bribes by the Brazilian company Odebrecht in order to take charge of public contracts for the construction of public works. FUNES analyzes the contracts, and during its launch, identified a huge number of contracts with corruption risks. Of these, several were investigated and transformed into published reports. FUNES is the first tool developed in Peru, and one of the first of its kind in Latin America, which analyzes millions of data, to grant a corruption risk score in public procurement. FUNES identified that between 2015 and 2018 the Peruvian State granted almost 20 billion dollars in risky contracts. These were delivered to a single bidder who had no competition and to companies created a few days before the contest. The amount represents 90 times the civil reparation that Odebrecht must pay for its acts of corruption. Other published reports identified acts of corruption in companies that sell milk for social programs.
The tool has a friendly interface for readers with several visualizations in which the reader can analyze the situation of public contracts in Peru. The open source tool has attracted the interest of the control and control entities of Peru, who have requested to share the methodology and possibilities so that they can implement it in their equipment. FUNES warns of risk in thousands of contracts. Therefore, and given the dimension of the findings, OjoPúblico established alliances with regional media to analyze and investigate some of the main cases. Everyone noticed the same thing: irregular public contracts that have now begun to be investigated by the authorities. The investigations continue.
技术/科技: Funes proviene de una familia de algoritmos denominados modelos lineales para combinar la información de 20 indicadores de riesgo, que fueron calculados a partir de 4 bases de datos. Un modelo lineal tiene la forma de un promedio ponderado: peso_1indicador_1 + peso_2indicador_2 + ... + peso_nindicador_n = riesgo de corrupción Para aprender estos pesos usualmente se utiliza un esquema de regresión, que consiste en intentar predecir la respuesta -que en este caso, sería la corrupción- a partir de variables relacionadas -como llamaremos a los indicadores de riesgo-. De esta manera, los pesos aprendidos para cada indicador son los que mejor ayudan a predecir la respuesta para todos los contratos analizados. Sin embargo, Funes usa una variante de este esquema porque la corrupción en contrataciones públicas -denominada nuestra variable respuesta- es un fenómeno no observable: tenemos seguridad de que los contratos que han sido descubiertos por los fiscalizadores fueron corruptos; pero los que no, no sabemos si están absolutamente limpios o aún no son descubiertos, porque pueden responder a sofisticados y esquemas de corrupción más complejos como sucede, por ejemplo, con el caso Odebrecht y Lava Jato. El método de Funes parte de un esquema de proxies de corrupción, propuesto por Mihaly Fazekas, investigador de la Universidad de Cambridge, y adecuado y p al contexto peruano de l. Un proxy es una variable estrechamente relacionada a la variable no observable. Funes usa dos proxies: 1) que un contrato haya tenido un único postor; 2) la proporción de concentración del presupuesto de una entidad que tiene cada contratista. Entonces, Funes es una combinación de dos modelos lineales, una regresión logística para el único postor y una regresión beta para la proporción de concentración. El resultado de este proceso es un índice de riesgo de corrupción para cada contrato: a más alto, mayor
作品最难完成的部分: The main challenges were related to the construction, access and quality of the data, the need for the team to learn new data analysis tools and the formation of a multidisciplinary team hitherto oblivious to journalistic research. In Peru there is no open data portal for hiring. For 7 months a script was developed and extracted data from a platform, which had blocked mass access through a captcha. The responsible entity blocked our IP to avoid downloading, forcing the team to reformulate the code to make extraction more efficient. To complete this information, 20 requests for access to information were also submitted. Another challenge was also the learning process on corruption theory, statistics and public procurement laws in Peru. We were not specialists in public bidding and there are 15 regulatory regimes. Meetings with experts were organized to know the process in detail, the processes were documented and each of the legal norms was analyzed.
Another of the challenges was also the definition of the concept of corruption that we were going to monitor and the model that we were going to use to develop the algorithm. Many papers were reviewed and interviews were conducted. In the end, the statistical model promoted by researcher Mihali Fazekas was chosen. The project left a journalistic team with robust knowledge in algorithms, R programming language, public contractings and predictability.
作品值得学习的地方: We learned that the fight against corruption from journalism requires incorporating into its traditional case-by-case methods and massive data analysis, tools with algorithmic models that allow it to anticipate corruption. For them, journalistic teams are required to go beyond spreadsheets and open refining, and learn relational analysis technologies and R., and at the same time learn to convene and work with mathematicians, statisticians, programmers and political scientists.
作品链接:
- https://ojo-publico.com/especiales/funes/
- https://ojo-publico.com/especiales/funes/rankings-de-riesgo.html
- https://ojo-publico.com/especiales/funes/metodologia.html
- https://ojo-publico.com/1499/proyecto-funes-riesgos-de-corrupcion-en-contratos-publicos
- https://ojo-publico.com/1331/familia-lechera-los-millonarios-contratos-del-grupo-niisa-con-el-vaso-de-leche
- https://knightcenter.utexas.edu/blog/00-21439-peruvian-investigative-site-ojo-publico-develops-algorithm-track-possible-acts-corrupt
- https://altec.lat/iniciativa-funes-corrupcion-en-peru-que-esta-haciendo-el-periodismo/
开放数据奖
得奖作品: TodosLosContratos.mx
机构: PODER
国家: 墨西哥
作者: Eduard Martín-Borregón, Martín Szyszlican, Claudia Ocaranza, Fernando Matzdorf, Félix Farachala, Marisol Carrillo, Ricardo Balderas and Isabela Granados.
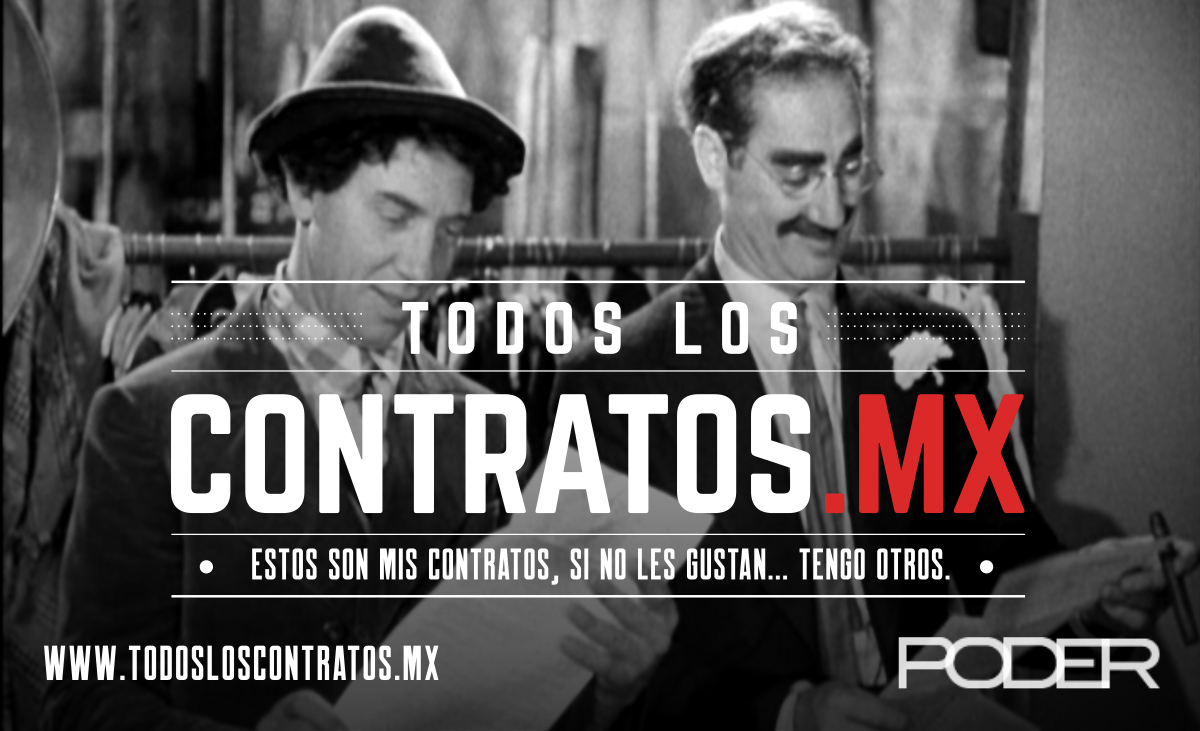
评审团评语: TodosLosContratos.mx is a massive open data endeavor. After cleaning and standardizing 4 million Mexico government contracts, the team built a website that provided top-line numbers and easy ways into this large database. But they didn’t stop there. They published all the data in a well-designed search engine, and a well-documented API. This project not only informed the general public but also empowered other journalists and researchers.
机构类别: 小型机构
发表日期: 20 Aug 2019
作品简介: TodosLosContratos.mx (All the contracts) is a data journalism project that has compiled almost 4 million public contracts made between 2001 and 2019 by the Mexican Federal Government. The project mixes journalistic reports that explain cases of corruption and bad practices in the mexican procurement system, with rankings based on algorithms specifically designed for the mexican by the team. The objective of the project is to promote accountability in the contracting process in Mexico, so we published all the data in QuiénEsQuién.wiki platform and API, opened the methodology of the analysis algorithms and published a guide on how to investigate with this tool.
作品效果与影响力: The publication of TodosLosContratos.mx together with the uploading of the data in QuiénEsQuién.Wiki has had three main impacts:
- Simplify the journalistic investigation of public contracts. The publication of the vast majority of contracts of the Mexican federal administration in a usable and reliable search engine has increase the productivity of the journalist, this has been expressed to us by journalists from Mexican outlets like Animal Político, Aristegui Noticias, El Universal, Cuestione, Proceso, among others, also local mexican online newspaper like Zona Docs, BI Noticias, Lado B or Cuestione, and International newspapers like AJ+ in spanish and El Faro (El Salvador).
- Promote the opening of public contracting data. Following our publication three government agencies have approached us to know how they can improve or upload new data to our platform. We have given them advice on how to improve their open data strategies; and once they publish we will update QuiénEsQuién.Wiki and our algorithmic analysis in TodosLosContratos 2020 edition.
- To increase the knowledge and interest of the citizens about the public procurement. As a result of the project, more people know how public contracting works and can easily consult it. Visits to the QuiénEsQuién.Wiki platform are increasing exponentially and every week we receive messages from people with doubts or clarifications about contracts or their participants.
技术/科技: A project of this complexity has several processes and key technologies:
- Data Import: Based in the free software Apache NiFi we have developed an importer and web scraper orchestrator. This modular software allows us to have a simple setting for reusable components like the data cleaning module or the data update module.
- Platform and API: QuiénEsQuién.Wiki is based on a mongoDB+node.js, all the data is hosted in a Kubernetes cluster of MongoDB databases and then exposed through a public API which is documented both in Spanish and English. Plus a model client in node js is usable with the NPM package registry. The website consumes the API and is compatible with desktop, tablets and mobile devices.
- Algorithmic analysis: Our "groucho" engine for analyzing open contracting data in the OCDS data standard. The engine is published with a GPL license, which makes it reusable and transparent. It's written in Node.JS.
- Data analysis: In order to fine tune the parameters of the algorithmic analysis engine we have combed through the data with the help of Kibana, an open source data visualization dashboard based on the ElasticSearch database engine, which helped us to quickly recognize patterns and detect deviations.
- Data visualization: Our data is nicely presented using custom designed web-based interactive graphs and maps using primarily the D3.js library.
作品最难完成的部分: For this project, our interdisciplinary team took the enormous task of automating the cleaning, compilation, transformation and analysis of 4 million contracts from 64 different tables of government-published data, a highlight of the hardest parts follows: - Data cleaning: The mexican government does not have a practice of unifying the name of the suppliers, neither they provide a unique identifier. Our "lavadora empresarial" software (also GPL) takes care of detecting duplicates with different spellings and other common errors, while avoiding to merge different but similar companies. For example, here's the page for Televisa in QuienEsQuien.wiki showing all the 23 different spellings of their name across 535 contracts. - Data transformation and compilation: Contracts from all sources are converted to the OCDS standard using specific mappings for each source, which can be very intricate with complex dependencies for the field values. 64 datasets are published in 5 different data structures, each of them requiring different pipelines in our Apache NiFi setup. These databases contain repeated contracts and several entries for the same contracting process which can only be compiled after they are transformed to OCDS standard. - Data analysis in an interdisciplinary team: Creating work tools which can be used by both journalists, programmers and analysts took several months and several long meeting until agreements were reached on the best way to capture specific malpractices in contracts or on why we could or couldn't perform specific evaluations with the available data.
作品值得学习的地方: Sharing our learned lessons is one of the main goals of the project, and encouraging others to emulate this kind of project. As we have said all of our projects are based in free software solutions, our own code is published in GPL licenses, all of our data and methodologies is published in CC-BY licenses. And all our reports are properly quote their sources. Plus we have documented the usage of our tools in Spanish and English, making everything we've done entirely reusable. We think the main takeaway is that it is possible to measure corruption based on public contracting data and we are starting to see the possibility of one day no longer relying on corruption perception surveys. Having a team that is committed to making bold assumptions and running deep journalistic analysis based in data was a key asset to accomplish our impact goals and to highlight our organization as one of the most advanced in the latinamerican region.
作品链接:
- https://www.todosloscontratos.mx/
- https://www.quienesquien.wiki/
- https://manualinvestigarcontrataciones.readthedocs.io/es/latest/#
- https://api.quienesquien.wiki/v2/docs/
- http://www.elclarinete.com.mx/mas-de-medio-millon-de-pesos-han-costado-visitas-de-amlo-a-aguascalientes/
- https://www.m-x.com.mx/al-dia/el-chef-de-las-estrellas-era-el-favorito-de-pena-nieto
学生和青年记者奖
得奖者: Rachael Dottle
机构: FiveThirtyEight.com, IBM Data and AI, freelance
国家: 德国
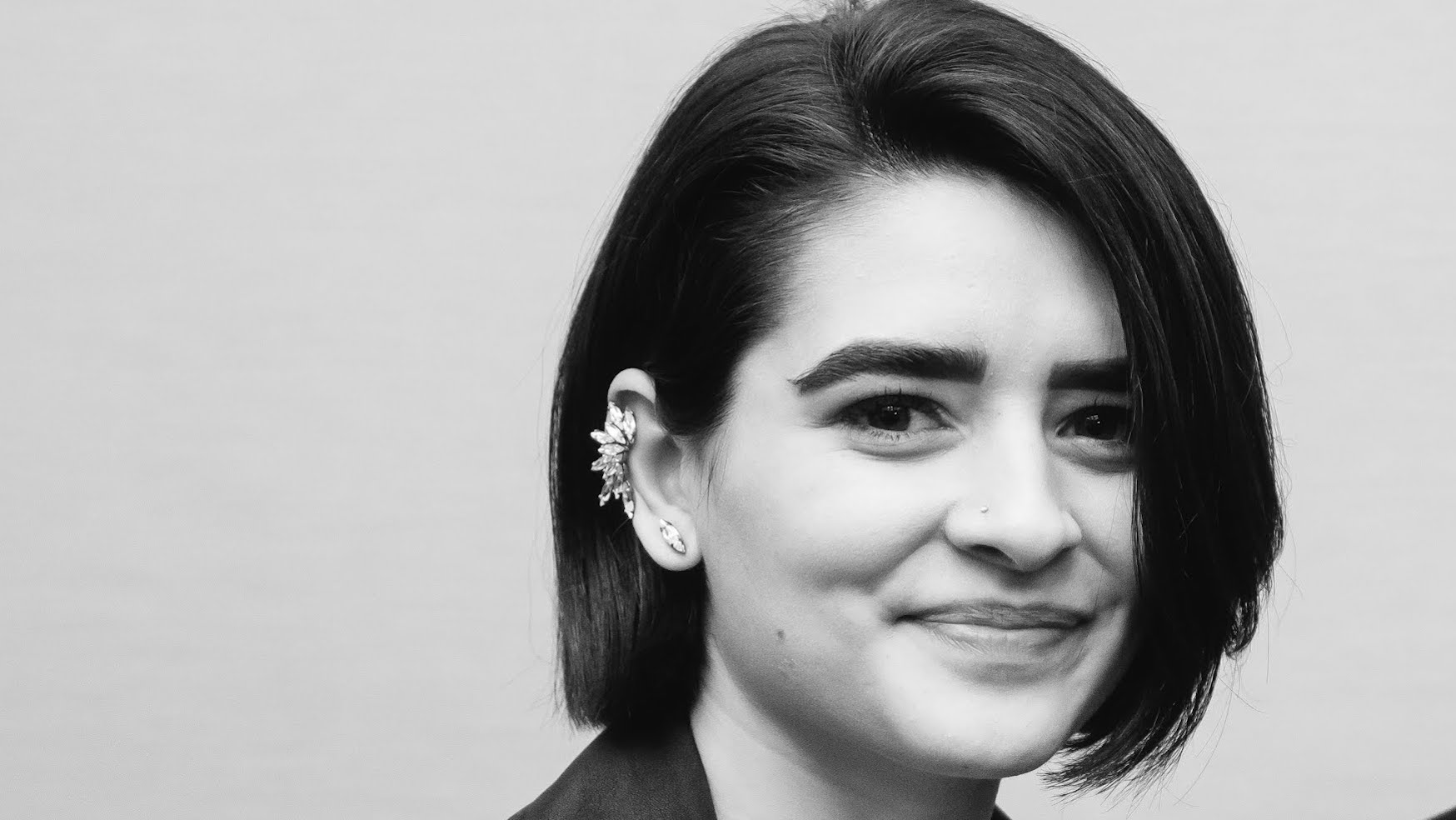
评审团评语: Rachel Dottle was named the Sigma’s Young Journalist in recognition of her work at fivethirtyeight.com. Rachel’s data reporting and visualizations are sophisticated and enlightening, bringing the reader along in exploration of the patterns in data. She upends preconceived notions by delving more deeply into data. An example is her work on where Republicans and Democrats live. “...just because Republicans aren’t winning in cities doesn’t mean that no Republicans live there. Much has been made of the country’s urban-rural political divide, but almost every Democratic city has Republican enclaves, especially when you think about cities as more than just their downtowns,” Rachel wrote. Then she let the reader explore exactly what she meant by digging into where the Republicans and Democrats were in metropolitan areas. She highlighted caveats in a delightfully conversational style. "You may notice that the map includes areas that you don’t consider urban. Take it up with the Census Bureau,” she wrote before explaining just how the Census has changed its definitions over time. And that was just one piece. Rachel has weighed in with a variety of political takes, from shifts in the suburbs rooted in her own family’s history to eliciting readers to weigh in with their own view on which political contenders have appeal with certain voting groups. And then there was a piece on the geographies of the most loyal college football fans. We know how deep the Rachel Dottle fan base is — and expect to see even more great work.
记者简介: As someone new to the field of data journalism, I stand on the shoulders of those editors, data visualization colleagues, and collaborators who mentored and encouraged me in journalism and in my work. As a data journalist I collect, analyze, organize, relay and visualize data. I attempt to do so in transparent and informative ways, while stretching my reporting and visualization skills. Data journalism is essential and ever-more important, and at the same time a field that continues to surprise and grow in exciting ways that make me proud of my work on the one hand, and excited to see and learn from the work of others.
作品简介: My portfolio is built around the work I've done as a data journalist at FiveThirtyEight of ABC News. As a data journalist, I work on small data visualization graphics that illustrate points being represented in data, as well as build stories around data discoveries and analyses. I've presented links to projects both large and small that demonstrate my reporting range, as well as the range of my ability to present meaning from data. I work with any tool available to me, including code, reporting and design. Each piece I publish is collaborative, which is the nature of data journalism, and which makes my work stronger, more transparent, and more accessible to readers at various levels of data literacy. I work to enlighten, inform and spark a tiny bit of joy, hopefully. I believe my portfolio and the breadth of my projects speak to my skills as someone early in their data journalism career.
作品链接:
- https://projects.fivethirtyeight.com/republicans-democrats-cities/
- https://projects.fivethirtyeight.com/democratic-candidate-appeal/
- https://projects.fivethirtyeight.com/college-football-ticket-sales/
- https://fivethirtyeight.com/features/a-tale-of-two-suburbs/
- https://projects.fivethirtyeight.com/2020-endorsements/democratic-primary/#all
- https://fivethirtyeight.com/features/the-movement-to-skip-the-electoral-college-just-passed-a-major-milestone/
回顾入围名单
以下是2020年Sigma数据新闻奖的入围名单:
最佳数据驱动报道(大型新闻机构),共10个
- Copy, Paste, Legislate
机构:USA TODAY, The Center for Public Integrity, The Arizona Republic (美国) -
The Troika Laundromat
机构: OCCRP(罗马尼亚), The Guardian(英国), Süddeutsche Zeitung(德国), Newstapa(韩国), El Periodico(西班牙), Global Witness以及另外17家伙伴媒体。 -
The Opioid Files
机构:The Washington Post (美国) -
In Brazil, at least 43 PMs are dismissed each day due to psychiatric disorders
机构:TV Globo (巴西) -
The Invisible Crime
机构:The Age, Sydney Morning Herald, Brisbane Times, WA Today (澳大利亚) -
53027条留言背后,网络树洞里绝望者的自救与互助
机构:澎湃, Sixth Tone (中国) -
The Quiet Rooms
机构:Chicago Tribune, ProPublica Illinois (美国) -
How top health websites are sharing sensitive data with advertisers
机构:Financial Times (英国) -
100 days of protests rock Hong Kong
机构:South China Morning Post (中国香港) -
Opportunity Zones
机构:ProPublica (美国)
最佳数据驱动报道(小型新闻机构),共6个
- Is There a Connection Between Undocumented Immigrants and Crime?
机构:The Marshall Project, published in collaboration with The New York Times's Upshot. (美国) -
Made in France
机构:DISCLOSE (法国) -
Shared Living: Mohammed gets fewer responses than Anna
机构:Addendum.org (奥地利) -
Privatized public spaces in Lima equals to 79 football fields
机构:Convoca.pe (秘鲁) -
Dying Homeless
机构:The Bureau of Investigative Journalism, members of the Bureau Local network (英国) -
Whose Home Is This? At Least 55,625 Properties Under the Hammer in Real Estate Auctions — and Counting: A data-driven research into housing financialization in Greece and the restructuring of the country by the markets, accompanied by an open dataset.
机构:AthensLive (English), The Press Project (Greek) (希腊)
最佳新闻应用,共11个
-
Which of these 2020 Democrats agrees with you most?
机构:The Washington Post (美国) -
Tuition Tracker
机构:The Hechinger Report (美国) -
Socios.red
机构:socios.red (阿根廷) -
The Election Game
机构:The Wire (印度) -
What inflation means for you
机构:Rappler (菲律宾) -
HOT DISINFO FROM RUSSIA (Topic radar)
机构:TEXTY.org.ua (乌克兰) -
Can I really afford to live in this place?
机构:Rádio Renascença (葡萄牙) -
The Language of Congress
机构:The Pudding (美国) -
Tiger Mum’s guide to getting your 6-year-old into the ‘right’ school
机构:Singapore Press Holdings - The Straits Times (新加坡) -
Cook spaghetti bolognese with us: We'll show you how climate-friendly your recipe is
机构:Neue Zürcher Zeitung (瑞士) -
Australia Talks news application
机构:Australian Broadcasting Corporation (澳大利亚)
最佳数据可视化报道(大型新闻机构),共8个
-
Key events from past four months of Hong Kong's anti-government protests
机构:South China Morning Post (中国香港) -
Drowning in plastic
机构:Reuters (新加坡) -
Self-driving cars: Who to save, who to sacrifice?
机构:Radio-Canada (加拿大) -
See How the World’s Most Polluted Air Compares With Your City’s
机构:The New York Times (美国) -
Why your smartphone is causing you ‘text neck’ syndrome
机构:South China Morning Post (中国香港) -
The Millions Who Left
机构:ZEIT ONLINE (德国) -
In a Notoriously Polluted Area of the Country, Massive New Chemical Plants Are Still Moving in
机构:ProPublica, The Times-Picayune and The Advocate (美国) -
How to Profit in Space: A Visual Guide
机构:The Wall Street Journal (美国)
最佳数据可视化报道(小型新闻机构),共9个
-
What people in Switzerland worry about
机构:SRF, Swissinfo (瑞士) -
The Unwelcomed
机构:Alhadaqa (埃及) -
The Gyllenhaal Experiment
机构:The Pudding (美国) -
Homicides overcome crisis of 2011
机构:La Data (墨西哥) -
Can you make AI fairer than a judge? Play our courtroom algorithm game
机构:MIT Technology Review (美国) -
Danish scam
机构:Pointer (KRO-NCRV) (荷兰) -
Como Bolsonaro votou nos últimos 20 anos na Câmara
机构:Nexo Jornal (巴西) -
Rafa Nadal's career in detail
机构:Mundo Deportivo (西班牙) -
Is Texas really going purple? Our Heat Index shows how competitive your district was — and is.
机构:Texas Tribune (美国)
最佳创新奖 (大型新闻机构),共6个
-
Winnti - Attacking the heart of the German industry
机构:Bayerischer Rundfunk (BR), Norddeutscher Rundfunk (NDR) (德国) -
AP DataKit: an adaptable data project organization toolkit
机构:The Associated Press (美国) -
Aggression Detectors: The Unproven, Invasive Surveillance Technology Schools Are Using to Monitor Students
机构:ProPublica, Wired (美国) -
Editorial dashboard to monitor ads spending on social media
机构:Sky News (英国) -
ADAM: The Automatic Data Article Machine
机构:RTL Nieuws (荷兰) -
Zones of Silence
机构:El universal (墨西哥)
最佳创新奖 (小型新闻机构),共7个
-
Chequeabot: Investing in technology that accelerates our impact
机构:Chequeado (阿根廷) -
RAPTOR
机构:FiveThirtyEight (美国) -
Visualizing the local – efficient data journalism for small newsrooms
机构:Nyhetsbyrån Siren (瑞典) -
SHELL SHOCKED
机构:The Intercept Brasil (巴西) -
Locked Out
机构:The Bureau of Investigative Journalism, members of the Bureau Local Network (英国) -
Funes: an algorithm to fight corruption
机构:OjoPúblico (秘鲁) -
When data meets politics
机构:Les Jours (法国)
开放数据奖,共14个
-
“Cocktail” of 27 different pesticides found in drinking water of 1 in every 4 municipalities
机构:Repórter Brazil, Public Eye, Agência Pública (巴西) -
Facebook Political Ad Collector – joint submission (The Globe and Mail and Quartz)
机构:The Globe and Mail, Quartz (加拿大) -
To Be Precise
机构:Charity Foundation 'Help Needed' (俄罗斯) -
BBC Shared Data Unit
机构:BBC Shared Data Unit (英国) -
Nonprofit Explorer Full-Text Search
机构:ProPublica (美国) -
Sold From Under You
机构:The Bureau of Investigative Journalism and HuffPost UK (英国) -
Sinapsis (Synapses): Fighting corruption with open data to discover connections between illegal companies that received public resources in Latin America
机构:Animal Político (墨西哥) -
MapMakoko
机构:Code for Africa, The Humanitarian OpenStreetMap, The Pulitzer Center on Crisis Reporting and Agency France Press (尼日利亚) -
绿网
机构:广州绿网环境保护服务中心 (中国) -
Database of Governments Officials Limos
机构:Balkan Investigative Reporting Network in Bosnia and Herzegovina (BIRN BiH) (波黑) -
The post-conflict numbers
机构:Rutas del Conflicto, Consejo de Redacción (哥伦比亚) -
Colaborabot
机构:Colaboradados (巴西) -
Fogo Cruzado
机构:Fogo Cruzado (巴西) -
TodosLosContratos.mx
机构:PODER (墨西哥)
学生和青年记者奖,共11位
-
Niko Kommenda
机构:The Guardian (英国) -
Cristina Suárez Vega
机构:El Confidencial (西班牙) -
Agnel Philip's 2019 portfolio
机构:The Arizona Republic (美国) -
G. Elliott Morris
机构:The Economist (美国) -
Somesh Jha
机构:Business Standard (印度) -
Rachael Dottle
机构:FiveThirtyEight.com (德国) -
Alessandro Sassoon
机构:Florida Today (美国) -
Ana Curic
机构:Balkan Investigative Reporting Network - BIRN (塞尔维亚) -
Luiz Fernando Toledo
机构:Fiquem Sabendo, Estadão, CNN Brasil, TV Globo (巴西) -
Pratheek Rebala
机构:The Center for Public Integrity (美国) -
Sharon Margriet Sumolang
机构:PT Narasi Citra Sahwahita (Narasi TV) (印尼)
Sigma数据新闻奖由Aron Pilhofer(美国天普大学)和Reginald Chua(路透社)发起,并得到Simon Rogers(谷歌)和Marianne Bouchart(HEI-DA,非盈利组织,致力于数据新闻领域发展)的大力支持。奖项由谷歌新闻计划(Google News Initiative)赞助,并由欧洲新闻中心(European Journalism Centre)旗下的DataJournalism.com网站协办。
实用信息
关于奖金
所有获奖者将获得一座奖杯,并获得赞助飞往意大利佩鲁贾参与2020年国际新闻节,(2020年4月1-5日)。每支获奖团队可委派2名成员参与国际新闻节,费用全免。
国际新闻节将设置专门的环节表彰获奖者。更重要的是,获奖者可以在此期间参与和领导数据新闻论坛、研讨和工作坊。
我们的使命
Sigma数据新闻奖的使命:
- 表彰全球最优秀的数据新闻作品;
- 围绕奖项建立活动和资源体系,让数据新闻业内及业外人士都可从中学有所得;
- 以奖项为契机,吸引、联结与赋能更多数据新闻业者,壮大数据新闻社群。
Sigma新闻奖团队的核心愿景是把全球的数据新闻工作者们联结在一起,彼此分享,彼此激励,并搭建一个长久甚至超越奖项本身的业界社群。
获奖者们将于2020年4月1日至5日的国际新闻节 (International Journalism Festival)期间,齐聚于风景秀丽的意大利历史名城佩鲁贾。届时,除了领奖,获奖者们还能参与和领导论坛、研讨和工作坊。在那里他们不仅能互相学习、分享,还将结识到很多优秀的数据新闻记者和有意从事数据新闻的记者。这样的业界联系将助益全球数据新闻记者之间的协作和共赢。
奖项组别
第一届Sigma数据新闻奖共设有6个组别,共9个奖项
1. 最佳数据驱动报道(分为小型与大型新闻机构)
参赛作品应极佳地运用数据作为调查性报道或解释性报道中坚实的组成部分。作品可以是独立报道,也可以是系列报道,但是都应基于数据和分析,采用与精确新闻、计算新闻或计算机辅助报道相关的技术。叙事、图表、互动、呈现也会被纳入评委的考量,但这一奖项侧重表彰通过数据的获取与分析,发现并讲述新闻故事的作品。
该奖项将分别授予小型新闻机构和大型新闻机构。
2. 最佳数据可视化作品(分为小型与大型新闻机构)
参赛作品应极佳地运用数据可视化的方式就一个公众关心的议题讲述新闻故事。数据可视化是否最好地传达新闻故事中的核心发现,是否清晰、准确、引人入胜以及采用合理正确的方法,是这一奖项主要考量的要素。评委亦会考虑数据分析和数据可视化所使用的技术,但首要因素仍然是可视化叙事本身。
该奖项将分别授予小型新闻机构和大型新闻机构。
3. 最佳创新奖(分为小型与大型新闻机构)
参赛作品应在拓展新闻创新边界上表现突出。在数据新闻生产过程中任何环节的创新都会得到认可,包括数据获取、清洗、分析、设计以及呈现。可以是已发表的数据新闻作品,也可以是开发该作品的工具、技术或程序。主要的考量因素是作品能反映数据新闻在制作程序或技术方面的显著进展,进一步推动数据新闻的发展。
该奖项将分别授予小型新闻机构和大型新闻机构。
4. 学生及青年记者奖
这个奖项表彰具卓越表现的学生或者27岁(含)以下的青年数据新闻记者(截至其作品发表时)。评委将通过所提交的作品考察参赛者在数据分析、使用、报道和呈现的质量和创新方面的表现。参赛者必须是作品的独立或者主要作者,每位参赛者最多可提交七个作品。
5. 开放数据奖
参赛作品致力于让数据开放,让其他记者、研究员和/或普通大众能获取与他们相关的数据。参赛作品可以是一个工具、一种技术,也可以是一个平台、一个已发表的新闻作品,无论哪种,只要是让与新闻相关的数据更开放或更容易获取,就可以角逐该奖项。作品的效果和影响力是考量的关键,参赛者应明确说明作品如何达到其效果或影响力。
6. 最佳新闻应用奖
一个出色的新闻应用必须让用户有能力在一个较大的数据库中发现自己的故事。参赛作品应是一个独立的新闻产品:它不需要借助附加的文字报道或讲解才能看懂,数据本身就是新闻作品。作品不是一个数据可视化或者数据驱动报道,尽管可以采用这两种手段。它应该是一个独立的站点或平台,提供用户工具让他们能在一个或多个数据库中筛选出与他们最为相关的资讯;它也可以是深度报道特定课题的站点,但仍然能让用户筛选数据,挖掘本身的故事。
参赛资格
- 作品必须发表于2019年。
- 作品必须通过在线报名提交。
- 作品必须于2020年2月5日23:59(美国东部时间,对应中国北京时间为2月6日12:59)前提交。
- 每一名参赛者可报名的作品无上限,但每一个作品只能报名一个奖项。如不确认作品该报哪一个奖项,请仔细阅读上述奖项说明并选取最合适的一个。在评选期间,如果评委发现某个作品更适合另一个奖项,他们会将该作品调整至合适的奖项下参与评选。
- 报名时需要明确说明作品参与的是大型还是小型新闻机构类别评选(如适用)。小型新闻机构应拥有不超过35名新闻记者(包括长期合作的自由记者和合同记者)或者相当规模(例如两名半职自由记者相当于一名全职记者)。
- 对于不符合这个规定但认为自己属于小型新闻机构的新闻组织,可由机构总编邮件至赛事经理Marianne Bouchart([email protected])申请特别处理。
- 多个新闻机构合作的作品须按大型新闻机构类报名。
- 报名参赛的新闻机构须允许Sigma奖主办方在比赛网站以及相关宣传材料上使用机构的名称、标志等素材。
其他事项
- Sigma奖的目标是在未来让参赛者能以不同的语言为作品报名,但由于2020年的报名截止日期已迫近,本届比赛只允许作品以英文提交报名资料。我们欢迎非英文作品,唯须提供尽可能详细的英文翻译。
- 评委们欢迎精简、用心铺陈,直击重点的报名内容。
评审团
评审团由Sigma奖的联席主席Reginald Chua和Aron Pilhofer领衔,并邀请到22位全球数据新闻领域的专家担任2020年度评委。他们是:
- Liliana Bounegru, 阿姆斯特丹大学(荷兰)
- Alberto Cairo, 迈阿密大学(美国)
- Feilding Cage, 路透社(美国)
- Sarah Cohen, 亚利桑那州立大学沃尔特·克朗凯特新闻及大众传播学院(美国)
- Kenneth Cukier, 《经济学人》(英国)
- Esra Dogramaci, 数字化策略顾问、编辑(卡塔尔)
- Steve Doig, 亚利桑那州立大学沃尔特·克朗凯特新闻及大众传播学院(美国)
- Amr Eleraqi, Info Times、阿拉伯数据新闻网络(埃及)
- Govindraj Ethiraj, IndiaSpend(印度)
- Joshua Hatch, 高等教育纪事报、慈善纪事报(美国)
- Scott Klein, ProPublica(美国)
- Darren Long, 南华早报(中国香港)
- Shazna Nessa, 华尔街日报(美国)
- Alastair Otter, 全球深度报道网、Passmark(南非)
- Cheryl Phillips, 斯坦福大学(美国)
- Paul Radu, OCCRP(罗马尼亚)
- Momi Peralta Ramos, La Nacion(阿根廷)
- Mariana Santos, Chicas Poderosas(巴西)
- Giannina Segnini, 哥伦比亚大学新闻学院(美国)
- Martin Stabe, 金融时报(英国)
- 邱悦, 彭博(美国/中国)
- 马金馨,联合国开发计划署(美国/中国)
初审团
主办方召集到10位全球数据新闻领域的专家协助初审2020年报名作品。
初审团队由赛事总监 Simon Rogers(谷歌)和赛事官郭史光庆率领。
评委还包括:
- Gurman Bhatia, 路透社(印度/新加坡)
- Eva Constantaras, Internews(希腊)
- Pinar Dag, Dağ Medya,土耳其数据新闻平台(土耳其)
- Peter Grensund, J++(瑞典)
- Mohammed Haddad, Al Jazeera (卡塔尔))
- Marco Hernandez, 路透社(新加坡)
- Marco Túlio Pires, 谷歌(巴西)
- Roberto Rocha, 加拿大广播公司(加拿大)
Sigma数据新闻奖团队
- Aron Pilhofer, 联席主席
- Reginald Chua, 联席主席
- Simon Rogers, 总监
- Marianne Bouchart, 经理
- 郭史光庆, 赛事官
- Paul Steiger, 顾问
联系我们
如有任何疑问,请邮件至Marianne Bouchart, [email protected]。
您可以上推特关注@SigmaAwards。
赛事进度
开放报名
首届Sigma数据新闻奖于2020年新年后开始接受报名,欢迎全球各地的作品积极参赛,在线报名。
第一届Sigma数据新闻奖的报名已于2020年2月5日结束。报名截止时间:2020年2月5日23:59(美国东部时间,对应中国北京时间为2月6日12:59)
揭晓奖项
在上述截止日期前仍可报名。入围与获奖名单已经于2020年2月公布。
进军佩鲁贾
组委会为获奖者安排前往佩鲁贾的行程,同时筹办Sigma数据新闻奖在国际新闻节中的专设环节。
欢庆时刻
2020年Sigma数据新闻奖的获奖者们将于4月1-5日在意大利佩鲁贾出席国际新闻节。新闻节期间将有专设的环节以表彰获奖作品,并让获奖者参与或领导数据新闻论坛、研讨和工作坊。
Sign up for our Conversations with Data newsletter
Join 10.000 data journalism enthusiasts and receive a bi-weekly newsletter or access our newsletter archive here.